Your Attribution Model Is Precise but Not Accurate - Here’s Why
Madeline Dault - Product Marketing Lead @ Haus
February 8, 2023
Does your brand search, last-click CPA look consistently incredible? Does that number actually indicate the true impact brand search is having on your business? Probably not. This is because last-click reporting is precise, but it is not accurate.
Marketers commonly confound accuracy and precision but the truth is, the two have very different meanings. Accuracy describes how close multiple measurements are to the truth on average whereas precision describes how close multiple measurements are to each other. This tends to be easier to visualize so take a look at the illustration below:
.png)
Now, when it comes to marketing measurement, it’s extremely important to understand if your ways of measuring impact are accurate, precise, neither, or both. Accuracy without precision is acceptable, but precision without accuracy leads your marketing decisions astray. In a perfect world, you'd have both. Let’s break down the most common ways marketers are measuring their impact.
Click Attribution
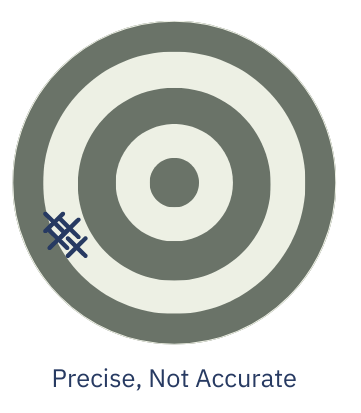
Whether you’re measuring your performance on a last-click basis or relying on a platform’s pixel attributed conversions, this form of measurement is precise but not accurate in telling you if the conversion was accretive to your business. It is precise in that platform reads are consistent and close to one another. However, it is not an accurate measurement of the incremental value this media brings to your business. For example, last-click attribution indicates the last step someone took before converting, but who’s to say that person wouldn’t have converted otherwise? It’s not a good gauge of incrementality and therefore is not accurate in demonstrating the business impact of the media.
Post Purchase Survey / HDYHAU

Companies often send surveys to individuals who have recently converted, asking “How did you hear about us?”. The respondents are required to reflect on their memory and recall the initial point of contact with the brand from a list of channels presented to them. Unfortunately, this data is neither accurate nor precise. Surveys are inaccurate because they rely on human memory which we know to be fallible. Furthermore, it presumes that the selected channel of introduction was also the driving force behind the decision to purchase, neglecting the potential influence of other channels. It’s not precise because one customer could respond in a variety of ways each time they answer the question depending on their purchase journey and their recollection. This form of attribution is susceptible to human error and should be approached with caution.
Media Mix Modeling / MMM

Media mix modeling is neither accurate nor precise. MMM has accuracy problems because brands tend to ramp up spend when going into peak time periods and ramp down in slower periods. Without experimentation, you can't be sure how much bias is caused by brands increasing spend into peak periods and decreasing spend into trough periods. If there is no spend variability, then there are no changes to be detected. It has precision problems because these models only look at aggregate daily KPI data while evaluating multiple media channels, so often there's just not much for the model to run on.
Geo-Testing Incrementality
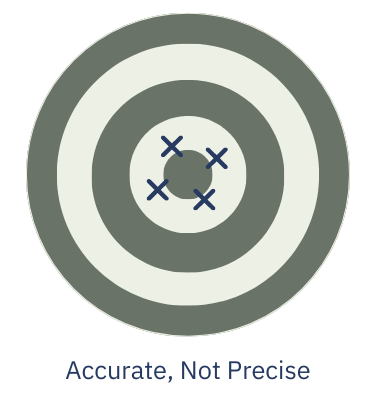
Geo-testing experiments are accurate but not always precise. Accuracy comes from creating random variation in the marketing and how it’s being delivered across the country. The experimental variation in itself is the control for accuracy. So this methodology is accurate but then, it’s a question of precision. To answer that question, you have to understand what techniques are being used to analyze the experiment.
Haus uses the geo-testing methodology (meaning it's accurate) and works to increase precision in a few ways. Haus utilizes synthetic control methods to ensure that the control regions are good comparables to the target regions. Rather than taking the average of the control regions, the synthetic control methods help create a weighted average making those regions a better comparison to the exposed regions. Additionally, once the data is in from the experiment, Haus’s model performs data cleaning for outliers in both the control and test groups. All of these tactics help to increase the precision of this kind of measurement.

If you’re ready for more accurate and precise marketing measurement, reach out to Haus here.