The Incrementality Blog
Insights and strategies for experiment-driven marketing measurement
Latest articles
Marketing Measurement: What to Measure and Why
.png)
This guide outlines the metrics, testing methods, and proven frameworks you can use to measure marketing effectiveness in 2025.
Why An Econometrics PhD Left Meta To Tackle Big Causal Questions at Haus
.png)
Senior Applied Scientist Ittai Shacham walks us through life on the Haus Science team and the diverse expertise needed to build causal models.
What You’re Actually Measuring in a Platform A/B Test

Platform creative tests may not meet the definition of a causal experiment, but they can be performance optimization tool within the bounds of the algorithm.
Beyond the Buzzwords: Why Transparency Matters in Incrementality Testing
.png)
Brands need to have complete information to make responsible decisions like their company depends on it.
Should I Build My Own MMM Software?
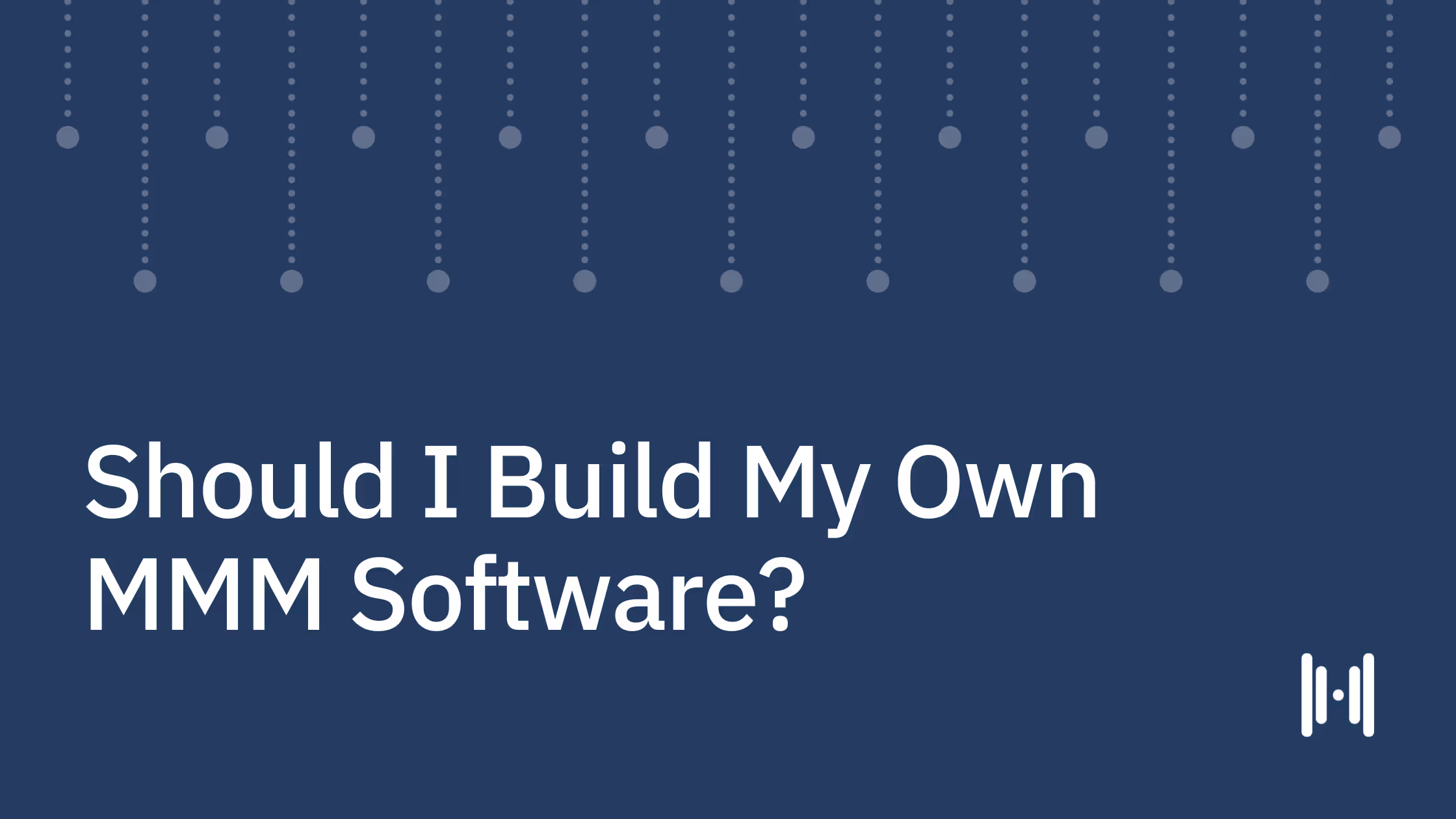
Let's unpack the pros and cons of building your own in-house marketing mix model versus working with a dedicated measurement partner.
Why An Analytics Expert Left Agency Life to Become Haus' First Measurement Strategist
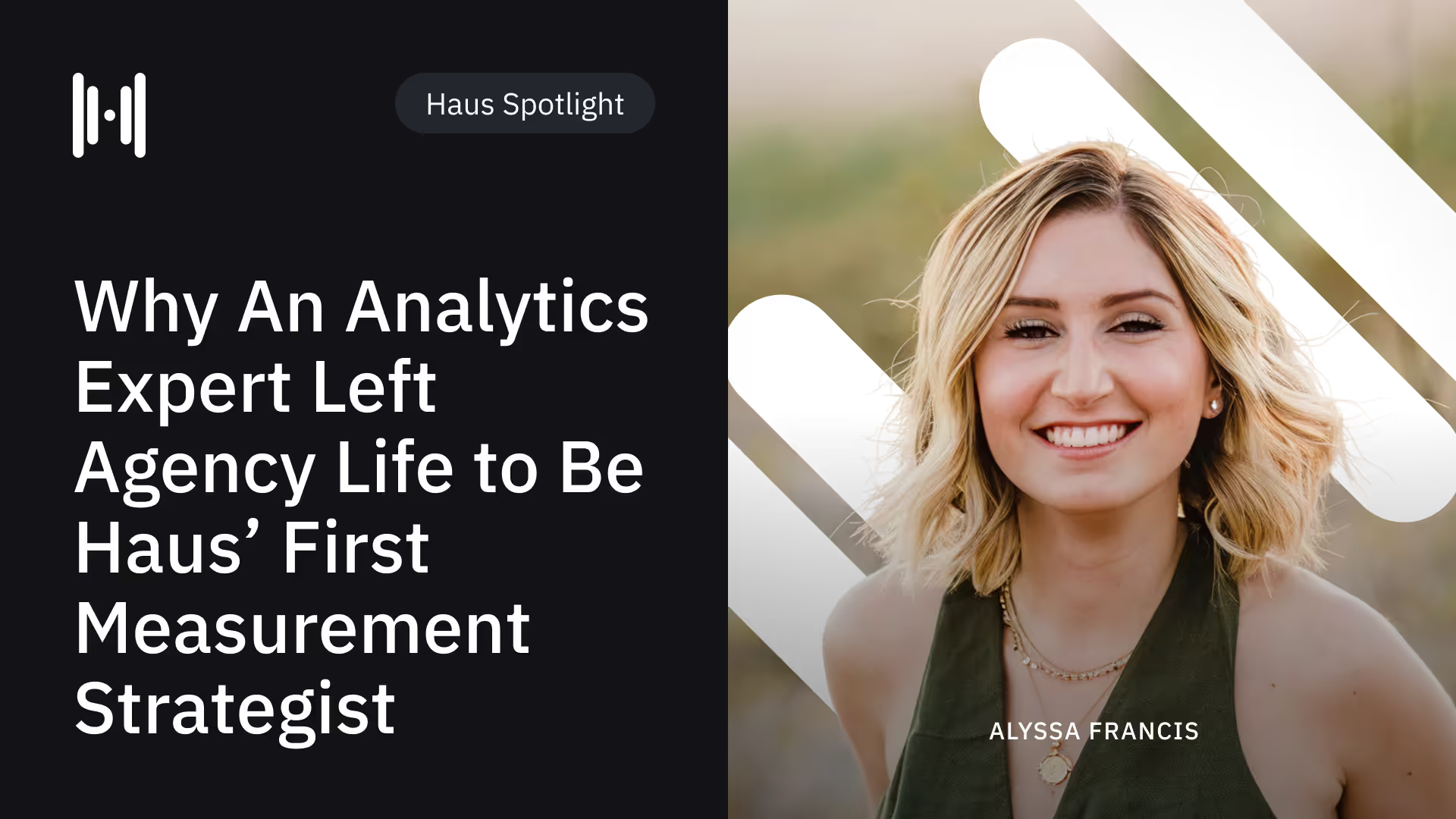
Measurement Strategy Team Lead Alyssa Francis sat down with us to discuss how she pushes customers to challenge the testing status quo.
Understanding Incrementality Testing
.avif)
Fuzzy on some of the nuances around incrementality testing? This guide goes deep, unpacking detailed examples and step-by-step processes.
MMM Software: What Should You Look For?
.avif)
We discuss some of the key questions to ask a potential MMM provider — and the importance of prioritizing causality.
How to Know If An Incrementality Test Result Is ‘Good’ – And What to Do About It
.avif)
Plus: What to do when a test result is incremental but not profitable, and a framework for next steps after a test.
Why A Leading Economist From Amazon Came to Haus to Democratize Causal Inference
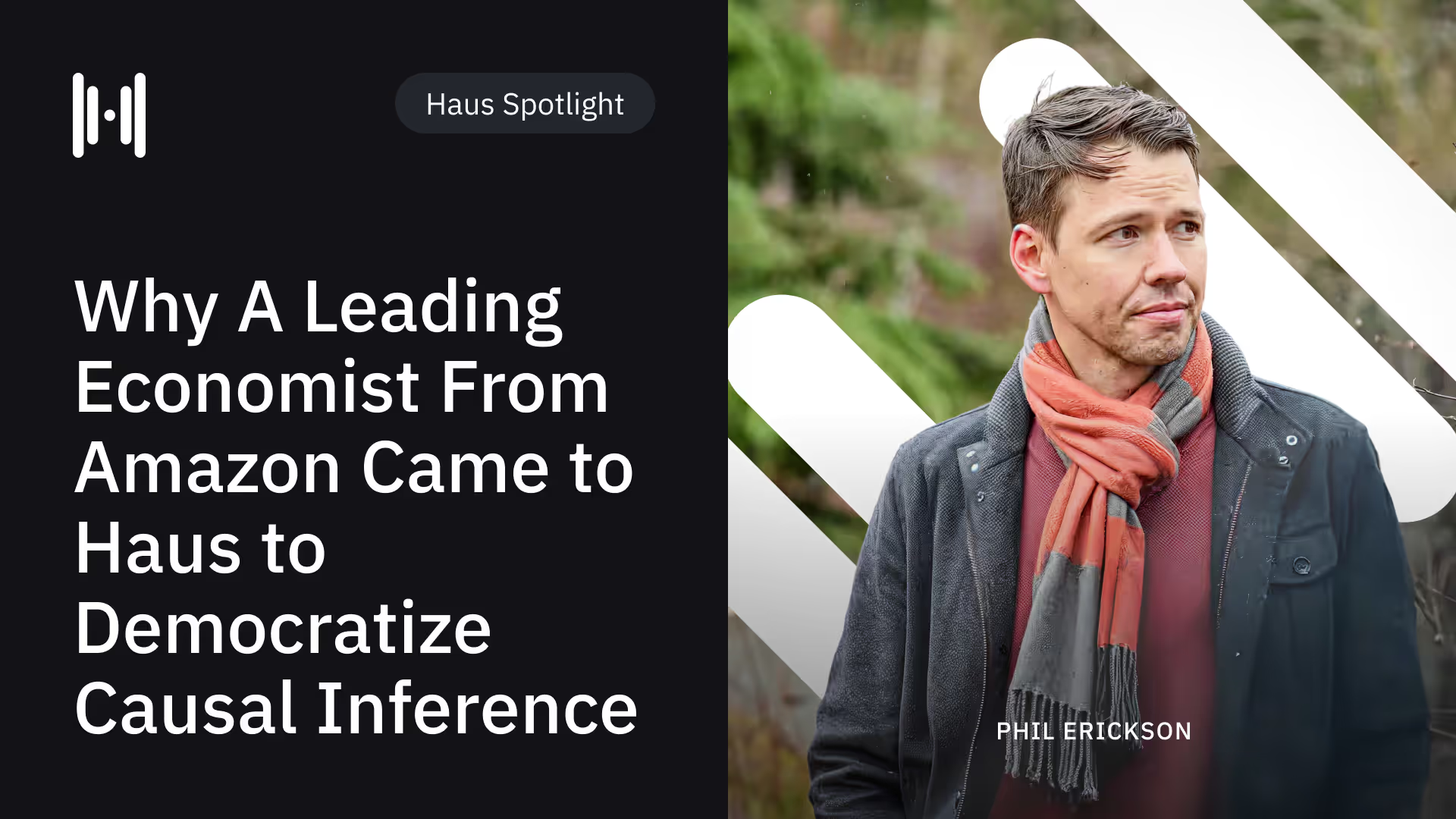
We sit down with Principal Economist Phil Erickson to talk about Haus’ “unhealthy obsession” with productizing causal inference.
Haus x Crisp: Measure What Matters in CPG Marketing
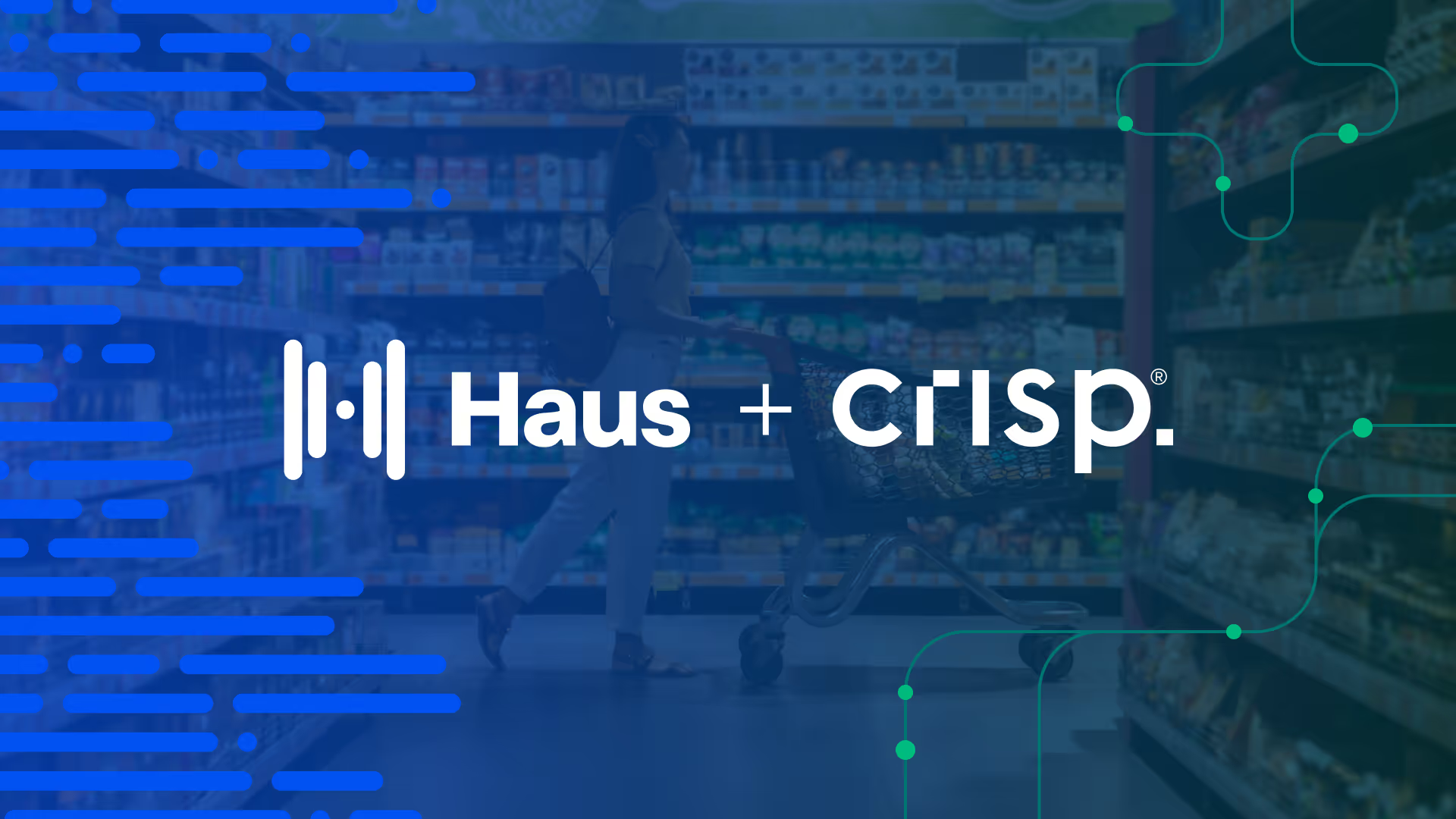
When real-time retail data meets incrementality testing, CPG brands can finally measure what’s working and optimize ad spend with confidence.
Why Magic Spoon’s Former Head of Growth Embraces Incrementality at Haus
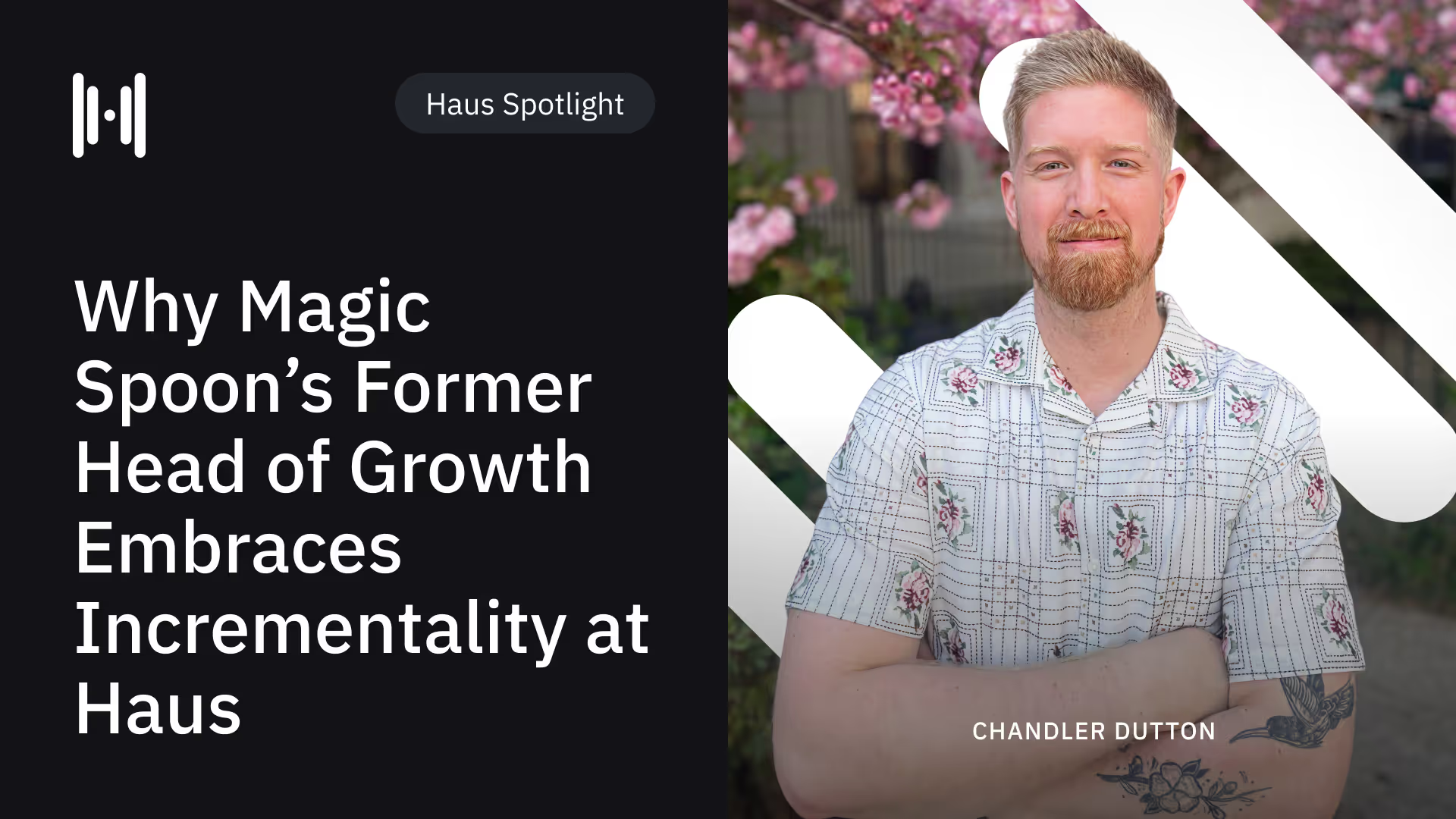
In our first episode of Haus Spotlight, we speak to Measurement Strategist Chandler Dutton about the in-the-weeds approach Haus takes with customers.
Do YouTube Ads Perform? Lessons From 190 Incrementality Tests
.avif)
An exclusive Haus analysis shows YouTube often delivers powerful new customer acquisition and retail halo effects that traditional metrics miss.
Getting Started with Causal MMM
.avif)
Causal MMM isn’t rooted in historical correlational data – it’s rooted in causal reality.
A First Look at Causal MMM
.avif)
Causal MMM is a new product from Haus founded on incrementality experiments. Coming 2025.
Would You Bet Your Budget on That? The Case for Honest Marketing Measurement

Acknowledging uncertainty enables brands to make better, more profitable decisions.
Incrementality: The Fundamentals
.avif)
Let's explore incrementality from every angle — what it is, what you can test, and what you need to get started.
Getting Started with Incrementality Testing

As the customer journey grows more complex, incrementality testing helps you determine the true, causal impact of your marketing.
Matched Market Tests Don't Cut It: Why Haus Uses Synthetic Control in Incrementality Experiments
.avif)
Haus’ synthetic control produces results that are 4x more precise than those produced by matched market tests.
Incrementality School, E6: How to Foster a Culture of Incrementality Experimentation
.avif)
Having the right measurement toolkit for your business is only meaningful insofar as your team’s ability to use that tool.
Geo-Level Data Now Available for Amazon Vendor Central Brands
.avif)
Vendor Central sellers – brands that sell *to* Amazon – can now use Haus to measure omnichannel incrementality.
How Does Traditional Marketing Mix Modeling (MMM) Work?
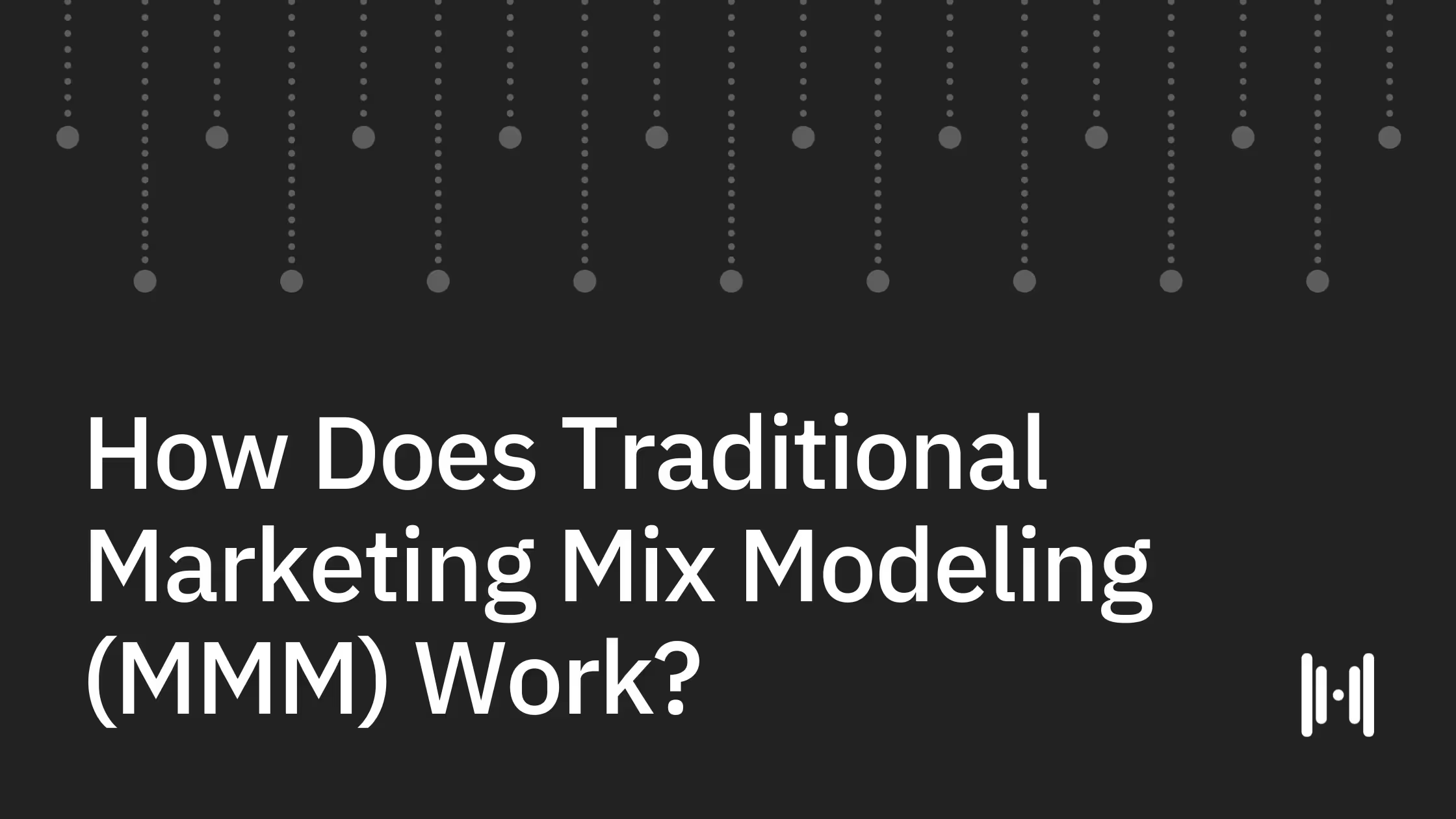
Traditional marketing mix modeling (MMM) often relies on linear regression to illustrate correlation, not causation.
2025: The Year of Privacy-Durable Marketing Measurement
.avif)
Haus incrementality testing doesn’t rely on pixels, PII, or other data that may be vulnerable to privacy regulations.
Meta Shares New Conversion Restrictions for Health and Wellness Brands
.avif)
Developing story: Starting in January 2025, some health and wellness brands may not be able to measure lower-funnel conversion events on Meta.
Incrementality School, E5: Randomized Control Experiments, Conversion Lift Testing, and Natural Experiments
.avif)
Sure, the title's a mouthful – but attributing changes in data (ex: ‘my KPI went up') to certain factors (ex: ‘we increased ad spend’) is hard to do well.
Incrementality Testing: How To Choose The Right Platform

Whether you’re actively evaluating incrementality platforms or simply curious to learn more, consider this checklist your first stop.
Incrementality School, E4: Who Needs Incrementality Testing?

As brands' marketing strategies grow in complexity, incrementality testing becomes increasingly consequential.
Incrementality School, E3: How Do Brands Measure Incrementality?
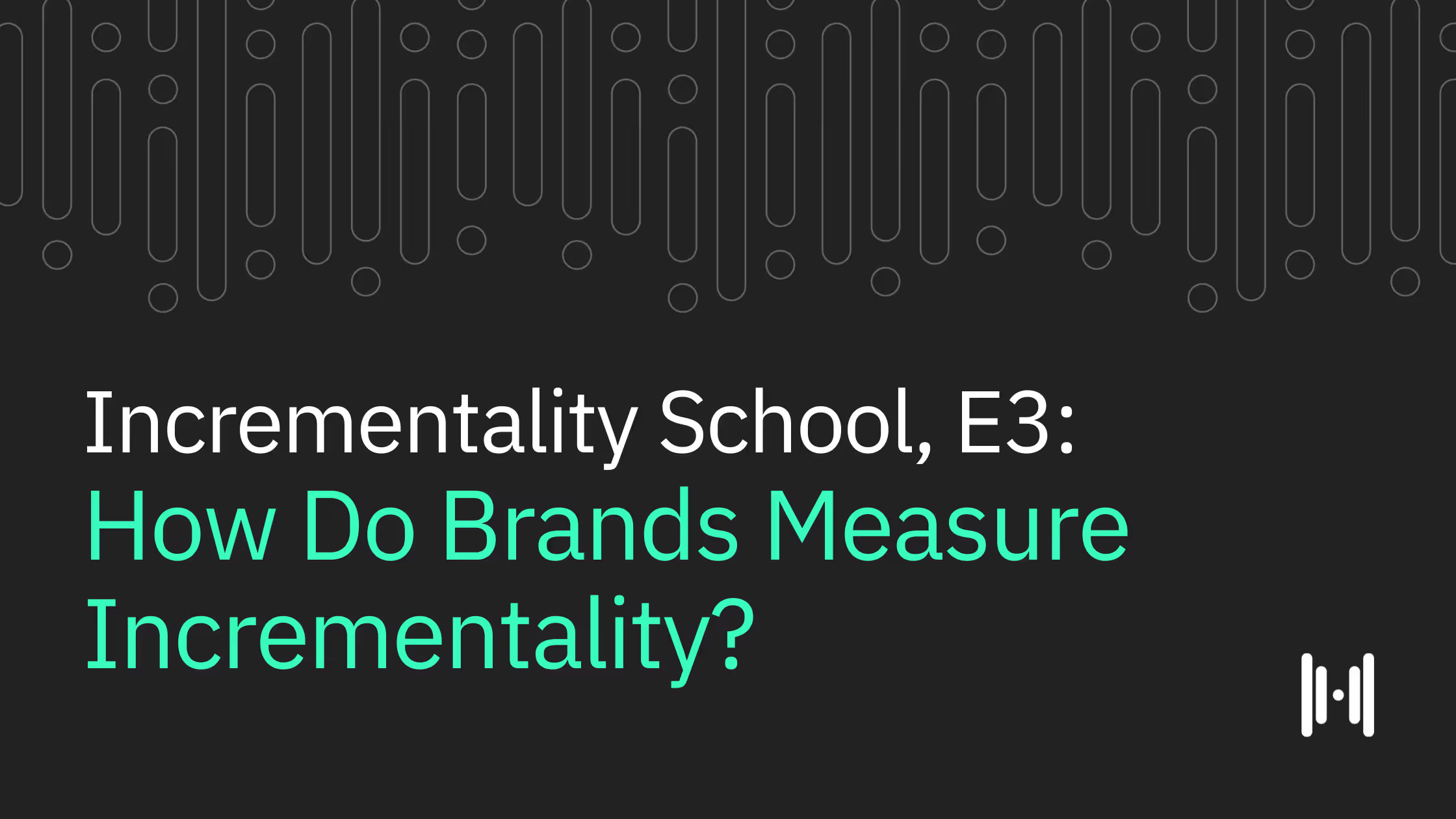
Traditional MTAs and MMMs won't measure incrementality – but geo experiments reveal clear cause, effect, and value.
Incrementality School, E2: What Can You Incrementality Test?

Haus’ Customer Marketing Lead Maddie Dault and Success Team Lead Nick Doren dive into what you can incrementality test – and why now's the time.
Incrementality School, E1: What is Incrementality?

To kick off our new Incrementality School series, three Haus incrementality experts weigh in describing a very fundamental concept.
Inside the Offsite: Why Haus?
.png)
At this year's offsite, we dove into why – of all the companies, options, and career paths out there – our growing team chose Haus.
Haus Named One of LinkedIn's Top Startups
.avif)
A note from Zach Epstein, Haus CEO.
Google Announces Plan to Migrate Video Action Campaigns to Demand Gen
.png)
The news leaves advertisers swimming in uncertainty — which is why it’s so important to test before the change.
Conversion Lag Insights: How Haus Tests Can Help Optimize Q4 Budgets
.avif)
Post-treatment windows offer a unique glimpse into the lingering impacts of advertising campaigns after they’ve concluded.
PMAX Experiments Revealed: Including vs. Excluding Branded Search Terms
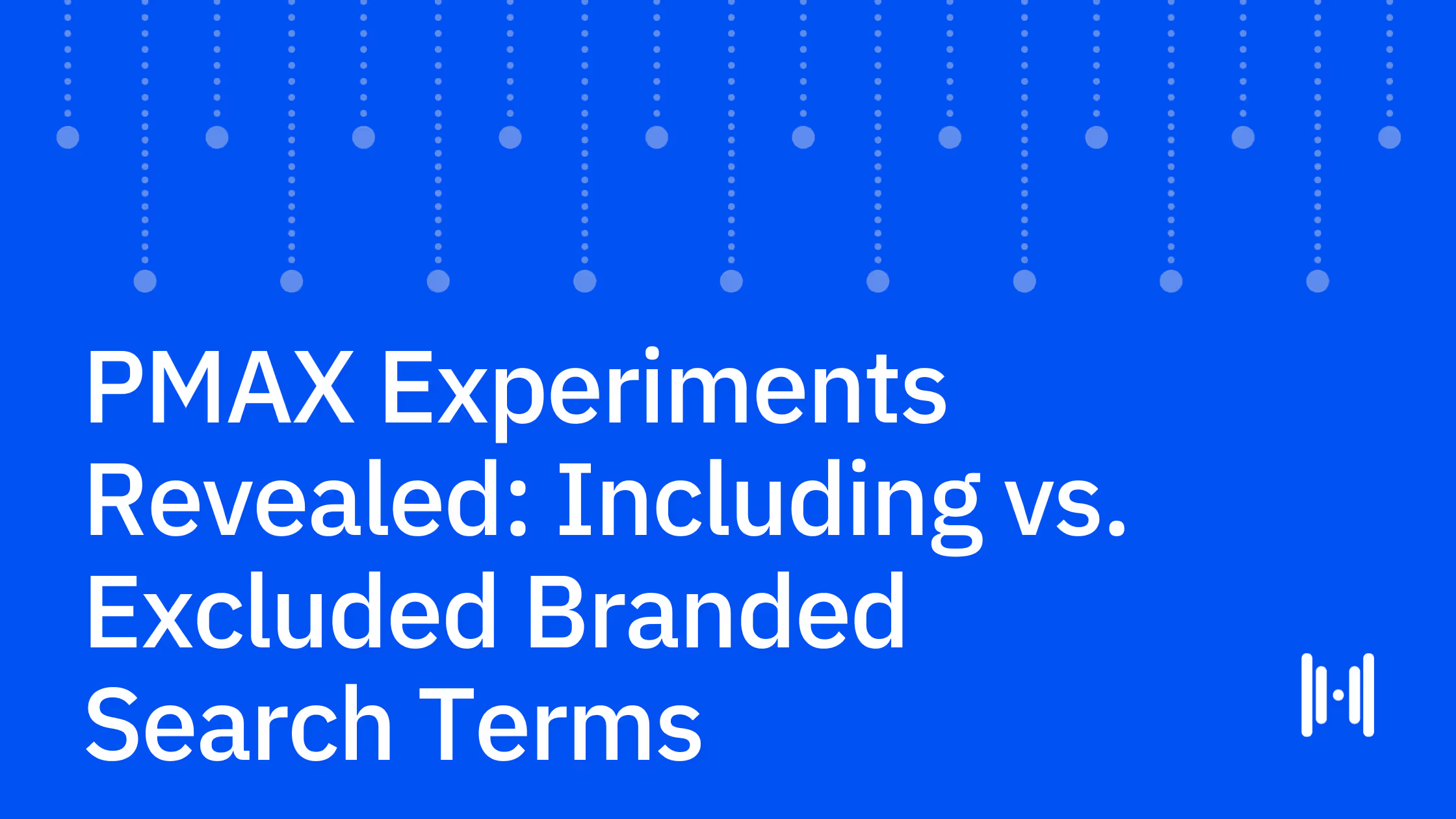
We analyzed experiments from leading brands to understand the incremental impacts of including vs. excluding branded terms in PMAX campaigns.
CommerceNext Session Recap: How Newton Baby Uses Incrementality Experiments to Maximize ROI

“We ran the test of cutting spend pretty significantly and it turns out a lot of that spend was not incremental,” says Aaron Zagha, Newton Baby CMO.
Introducing Causal Attribution: Your New Daily Incrementality Solution

Causal Attribution syncs your ad platform data with your experiment results to provide a daily read on which channels drive growth.
Haus Announces $20M Raise Led by 01 Advisors
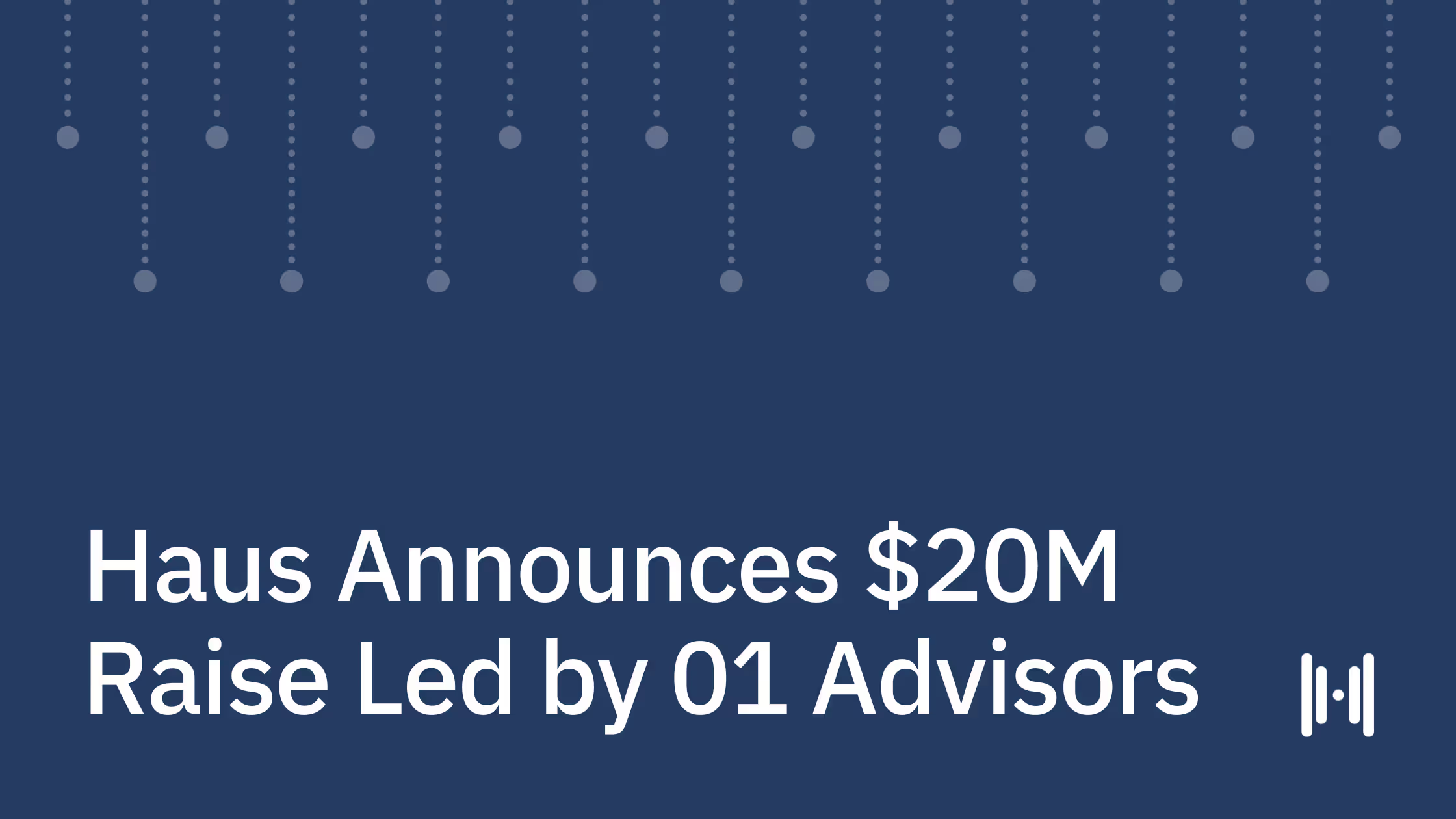
With this additional support, Haus is well-positioned to deepen our causal inference capabilities and announce a new product: Causal Attribution.
3 Ways to Perfect Your Prime Day Marketing Strategy
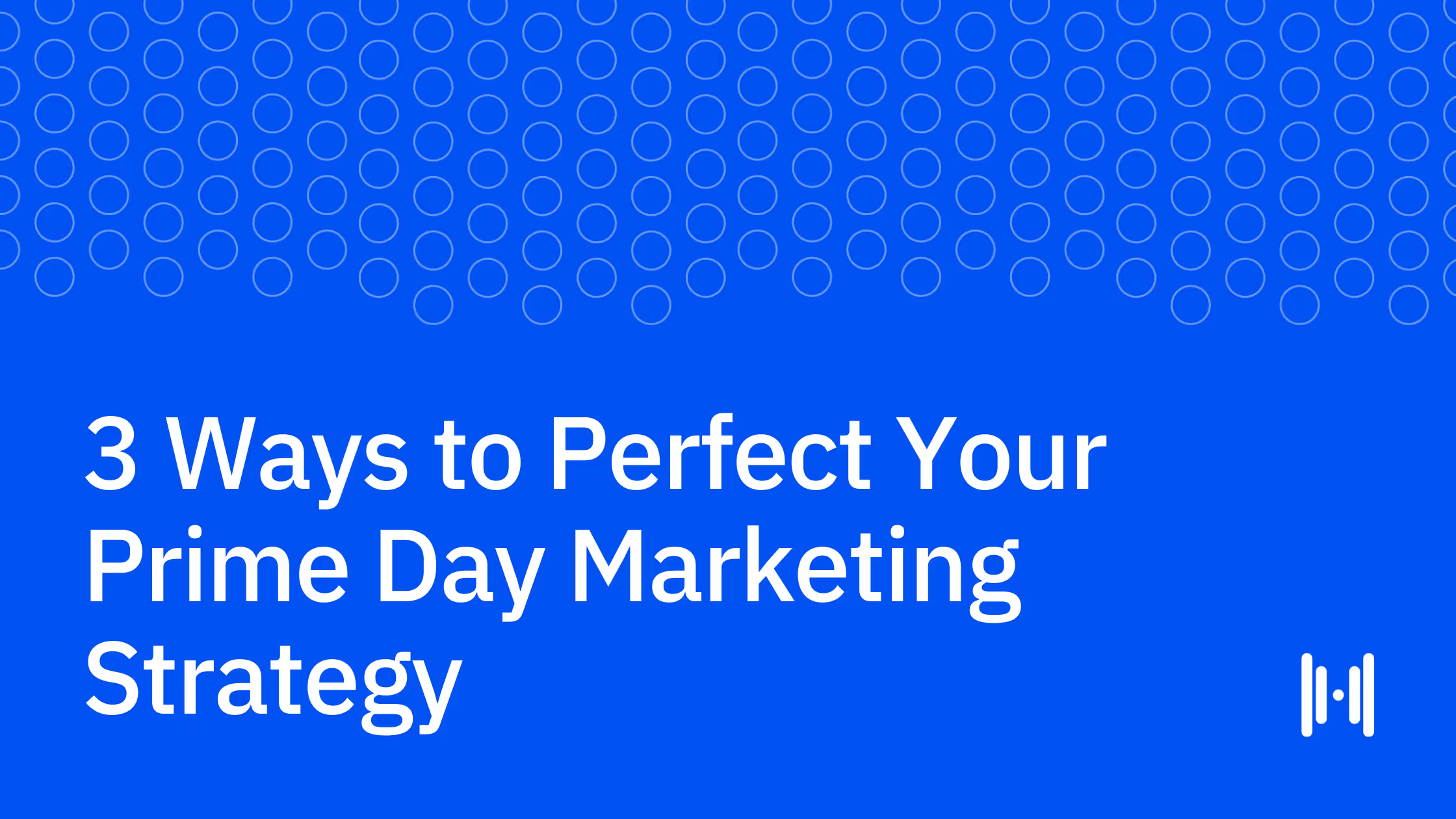
Think Amazon ads are the only effective marketing channel for Prime Day? Think again.
Maximize Your Q4 Growth With 4 High-Impact, Low-Risk Tests

Not testing during your busy season may be costing you more than you think.
Why Maturing Direct to Consumer Brands Need to Run Incrementality Tests

The media strategy that gets DTC brands from zero to one does not get them from one to ten.
5 Signs It’s Time to Invest in Incrementality
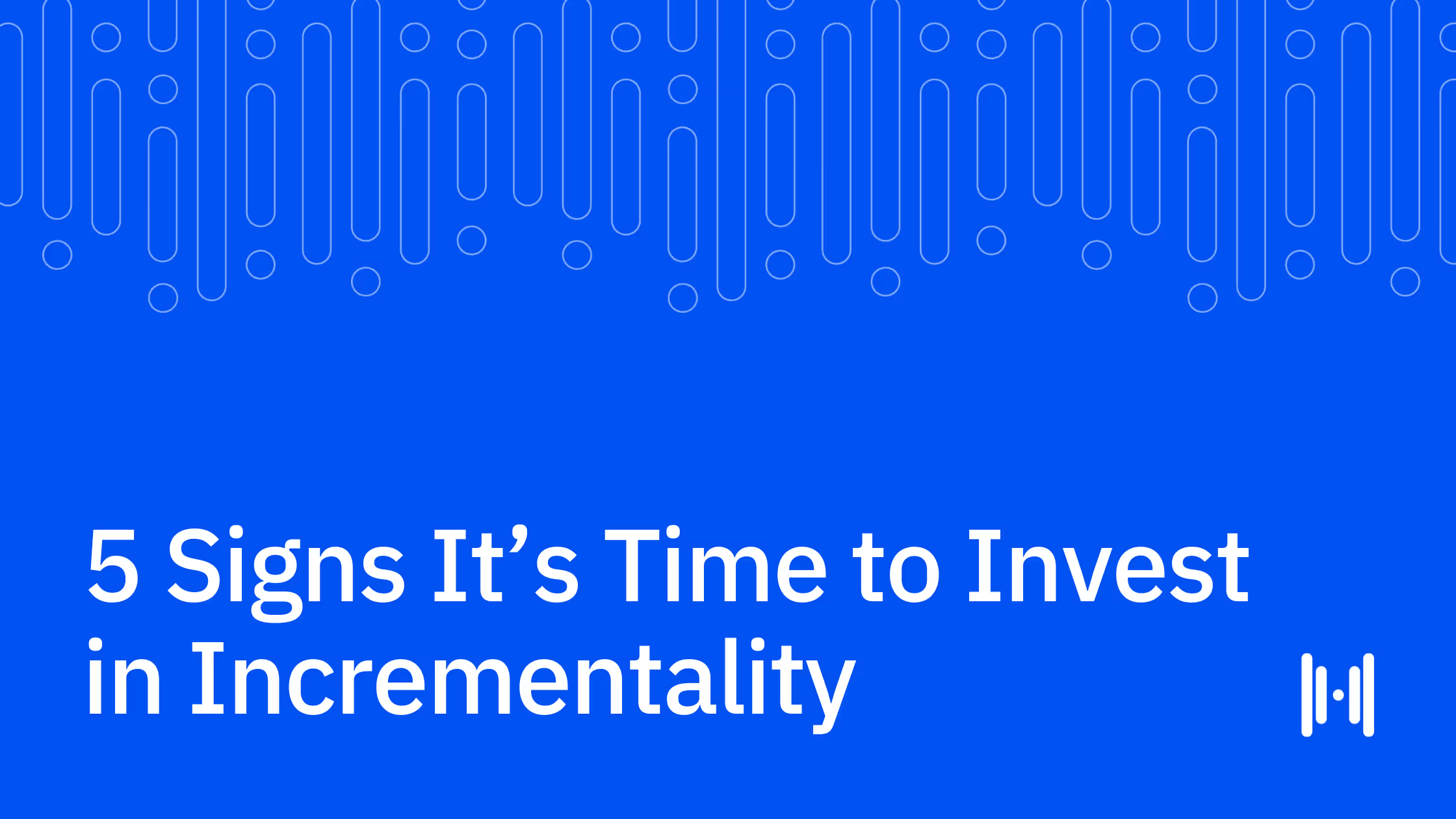
5 common signs that indicate it is definitely time to start investing in incrementality.
$17M Series A, Led by Insight Partners
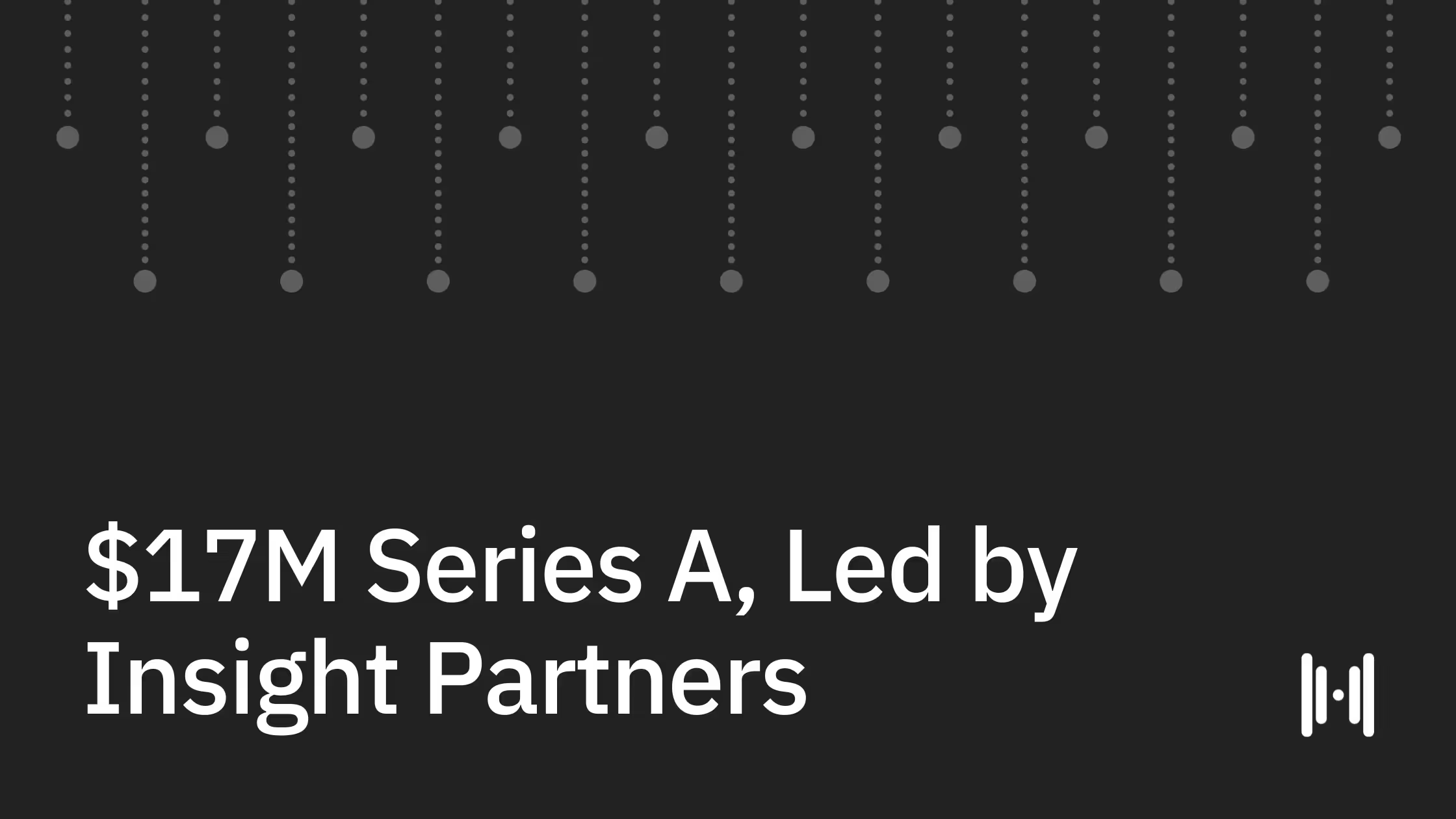
Haus raises $17M Series A led by Insight Partners to build the future of growth intelligence.
Why Meta's “Engaged Views” Is a Distraction, Not a Solution

While additional data can be useful, we must question whether this new rollout is truly a solution or merely another diversion.
Why You Need a 3rd Party Incrementality Partner

Are you stuck wondering if you should be using 3rd party incrementality studies, ad platform lift studies, or trying to design your own? Find out here.
iOS 17 Feels Like iOS 14 All Over Again. What It Means for Growth Marketing…And Does It Matter Anymore?

A single press release vaguely confirmed that Apple will continue its assault on user level attribution. Here, I unpack what I think it means for growth marketing.
How Automation Is Transforming Growth Marketing

As platforms force more automation, the role of the media buyer is evolving. Read on to learn what to expect and what levers are left to pull.
Statistical Significance Is Costing You Money

It is profitable to ignore statistical significance when making marketing investments.
The Secret to Comparing Marketing Performance Across Channels

While incrementality is better than relying on attribution alone, comparing them as-is is challenging. Thankfully, there’s a better way to get an unbiased data point regardless of the channel.
Your Attribution Model Is Precise but Not Accurate - Here’s Why

Learn which common marketing measurement tactics are accurate, precise, neither or both.
How to Use Causal Targeting to Save Money on Promotions

Leverage causal targeting to execute promotions that are actually incremental for your business.
Are Promotions Growing Your Business or Losing You Money?

Promotions, despite their potential power and ubiquity, are actually hard to execute well.
User-Level Attribution Is Out. Geo-Testing Is the Future.
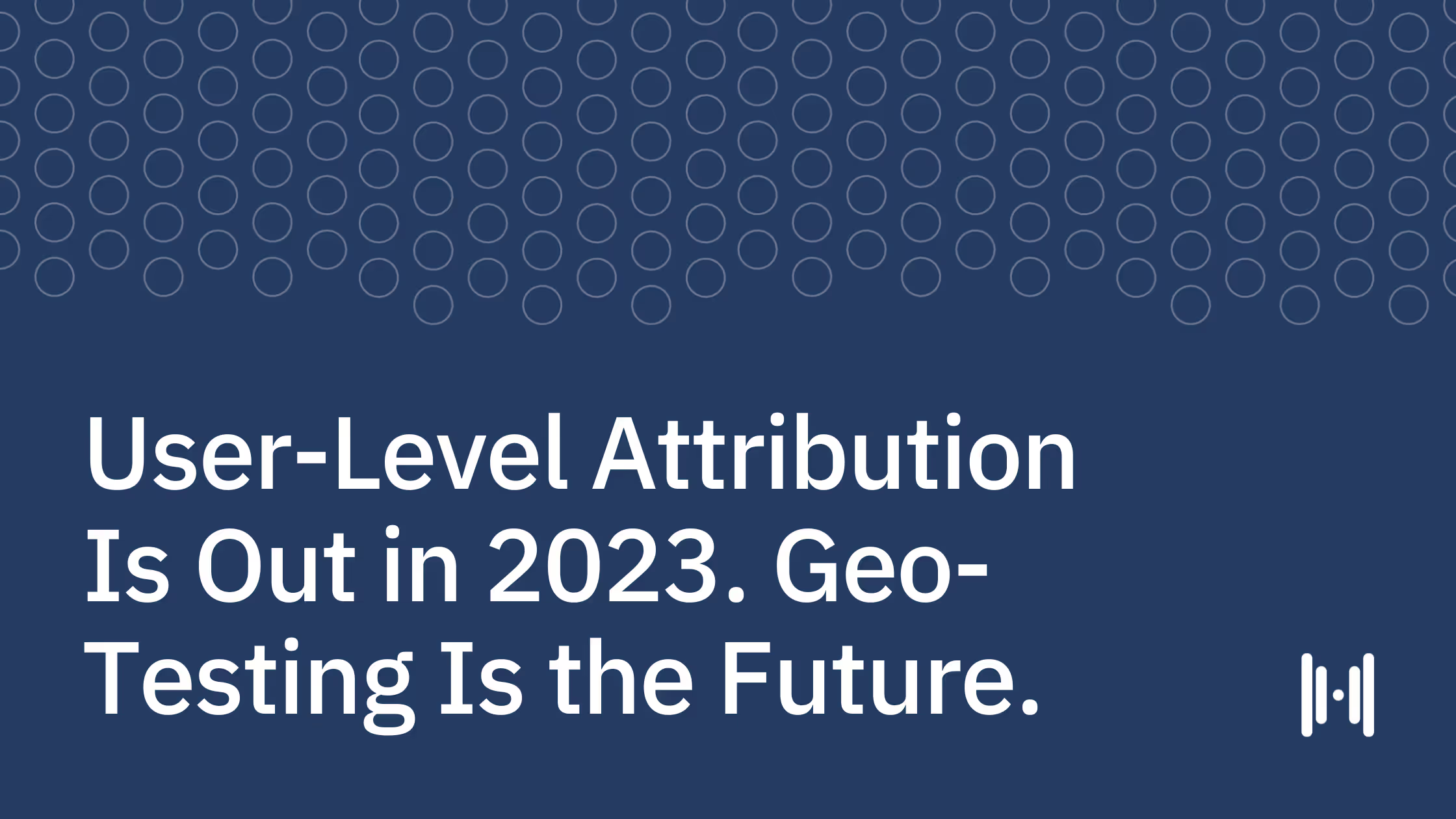
Geotesting is a near-universal approach for measuring the incremental effects of marketing across both upper and lower funnel tactics.
The Haus Viewpoint

We are building Haus to democratize access to world-class decision science tools.
From the Lab
The latest experiment results and thought leadership from the Haus team.
Beyond the Buzzwords: Why Transparency Matters in Incrementality Testing
.png)
Brands need to have complete information to make responsible decisions like their company depends on it.
Do YouTube Ads Perform? Lessons From 190 Incrementality Tests
.avif)
An exclusive Haus analysis shows YouTube often delivers powerful new customer acquisition and retail halo effects that traditional metrics miss.
Would You Bet Your Budget on That? The Case for Honest Marketing Measurement

Acknowledging uncertainty enables brands to make better, more profitable decisions.
Matched Market Tests Don't Cut It: Why Haus Uses Synthetic Control in Incrementality Experiments
.avif)
Haus’ synthetic control produces results that are 4x more precise than those produced by matched market tests.
2025: The Year of Privacy-Durable Marketing Measurement
.avif)
Haus incrementality testing doesn’t rely on pixels, PII, or other data that may be vulnerable to privacy regulations.
Conversion Lag Insights: How Haus Tests Can Help Optimize Q4 Budgets
.avif)
Post-treatment windows offer a unique glimpse into the lingering impacts of advertising campaigns after they’ve concluded.
PMAX Experiments Revealed: Including vs. Excluding Branded Search Terms
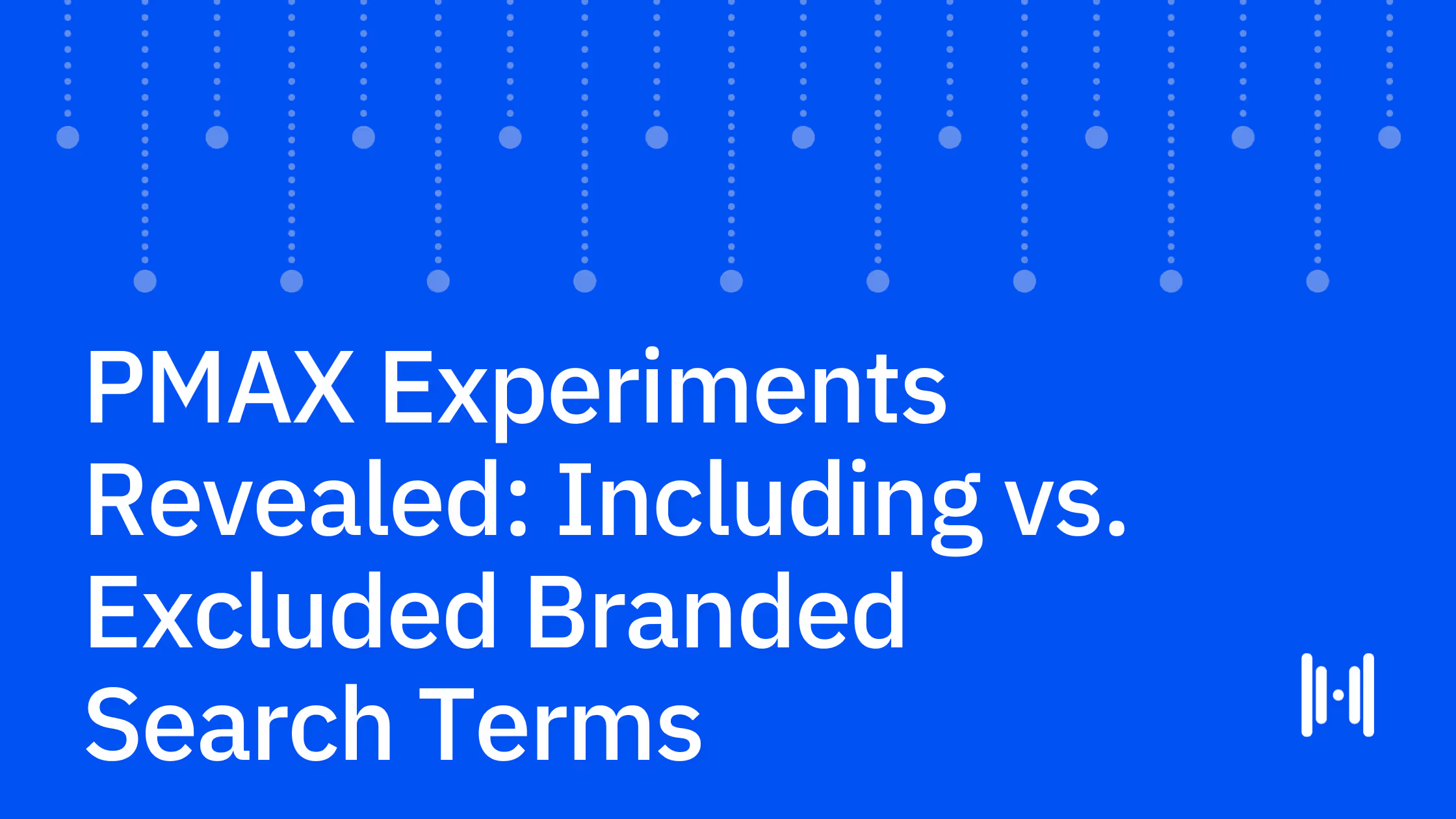
We analyzed experiments from leading brands to understand the incremental impacts of including vs. excluding branded terms in PMAX campaigns.
CommerceNext Session Recap: How Newton Baby Uses Incrementality Experiments to Maximize ROI

“We ran the test of cutting spend pretty significantly and it turns out a lot of that spend was not incremental,” says Aaron Zagha, Newton Baby CMO.
Statistical Significance Is Costing You Money

It is profitable to ignore statistical significance when making marketing investments.
Incrementality School
Incrementality School, E1: What is Incrementality?

To kick off our new Incrementality School series, three Haus incrementality experts weigh in describing a very fundamental concept.
Incrementality School, E2: What Can You Incrementality Test?

Haus’ Customer Marketing Lead Maddie Dault and Success Team Lead Nick Doren dive into what you can incrementality test – and why now's the time.
Incrementality School, E3: How Do Brands Measure Incrementality?
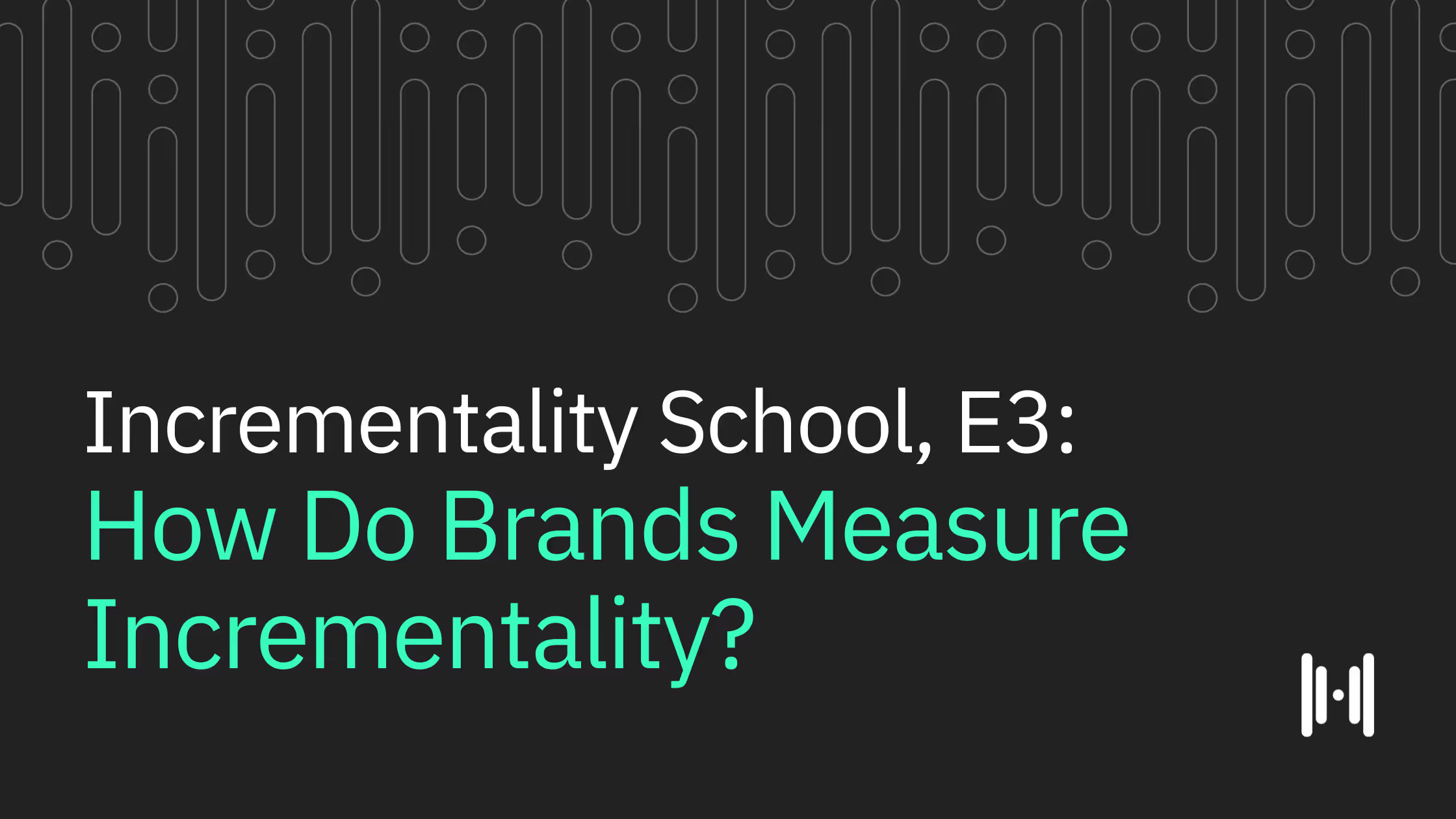
Traditional MTAs and MMMs won't measure incrementality – but geo experiments reveal clear cause, effect, and value.
Incrementality School, E4: Who Needs Incrementality Testing?

As brands' marketing strategies grow in complexity, incrementality testing becomes increasingly consequential.
Incrementality School, E5: Randomized Control Experiments, Conversion Lift Testing, and Natural Experiments
.avif)
Sure, the title's a mouthful – but attributing changes in data (ex: ‘my KPI went up') to certain factors (ex: ‘we increased ad spend’) is hard to do well.
Incrementality School, E6: How to Foster a Culture of Incrementality Experimentation
.avif)
Having the right measurement toolkit for your business is only meaningful insofar as your team’s ability to use that tool.
Inside Haus
Learn a bit more about who we are and what we do.
Why An Econometrics PhD Left Meta To Tackle Big Causal Questions at Haus
.png)
Senior Applied Scientist Ittai Shacham walks us through life on the Haus Science team and the diverse expertise needed to build causal models.
Why An Analytics Expert Left Agency Life to Become Haus' First Measurement Strategist
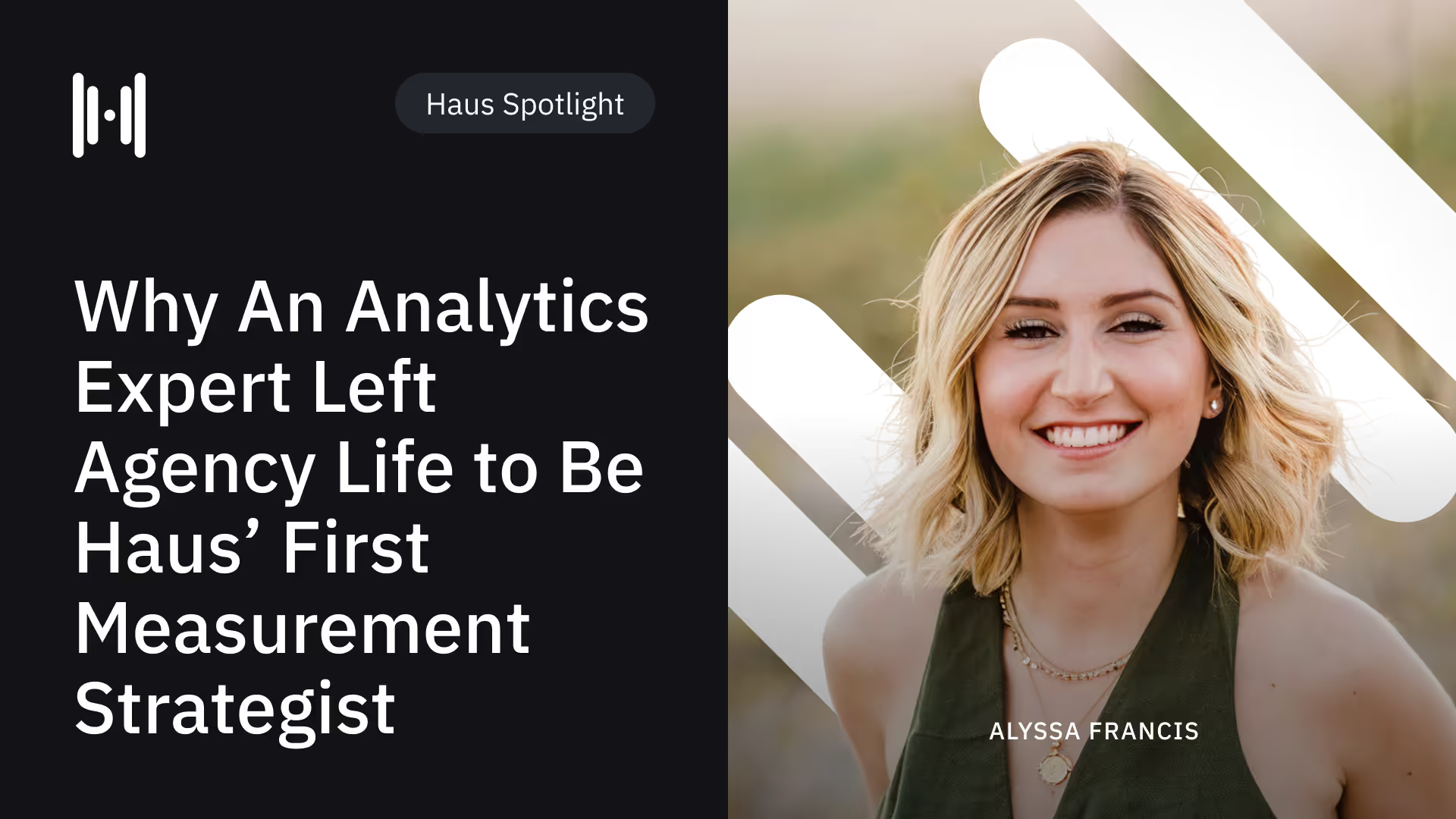
Measurement Strategy Team Lead Alyssa Francis sat down with us to discuss how she pushes customers to challenge the testing status quo.
Why A Leading Economist From Amazon Came to Haus to Democratize Causal Inference
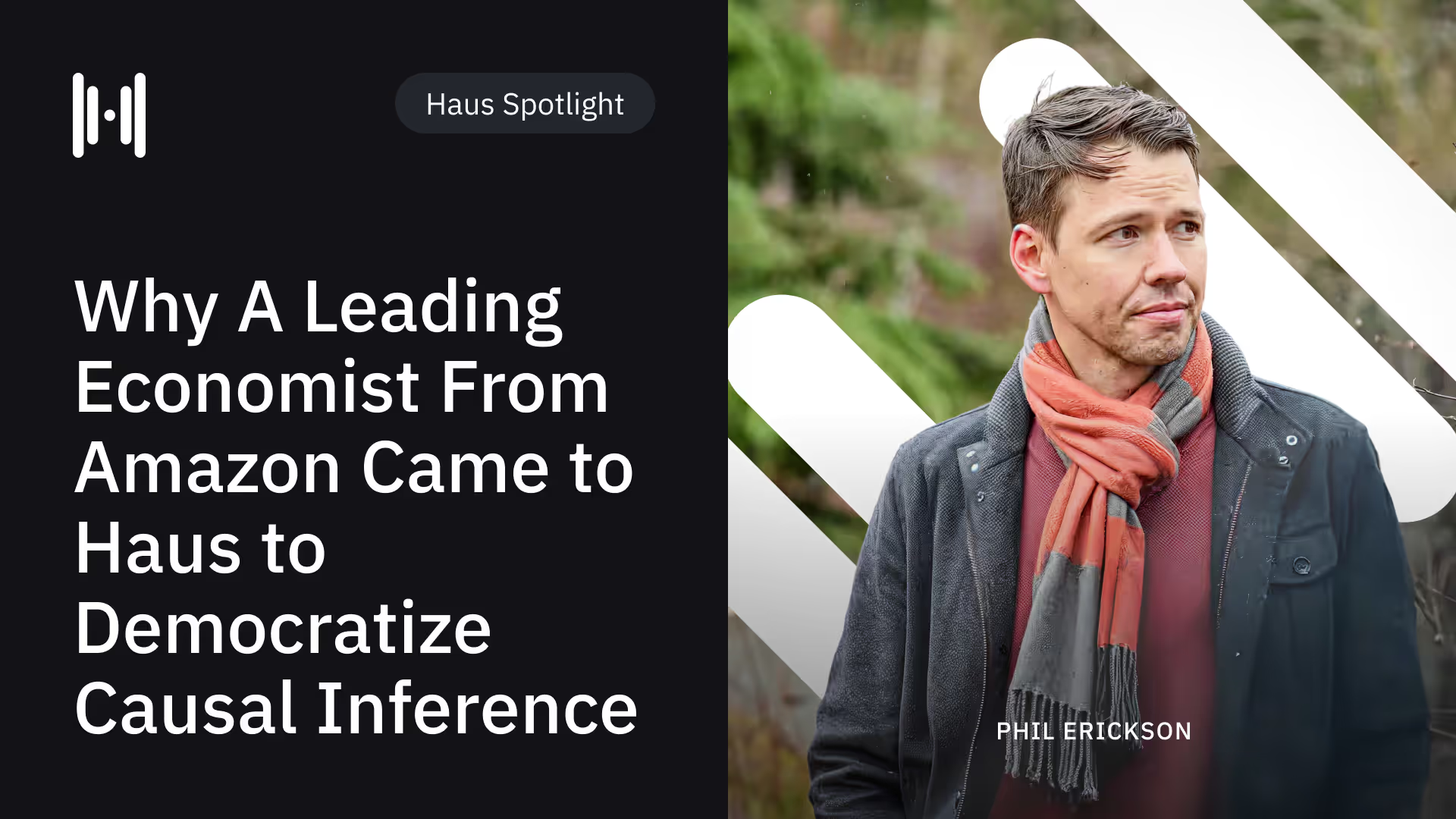
We sit down with Principal Economist Phil Erickson to talk about Haus’ “unhealthy obsession” with productizing causal inference.
Why Magic Spoon’s Former Head of Growth Embraces Incrementality at Haus
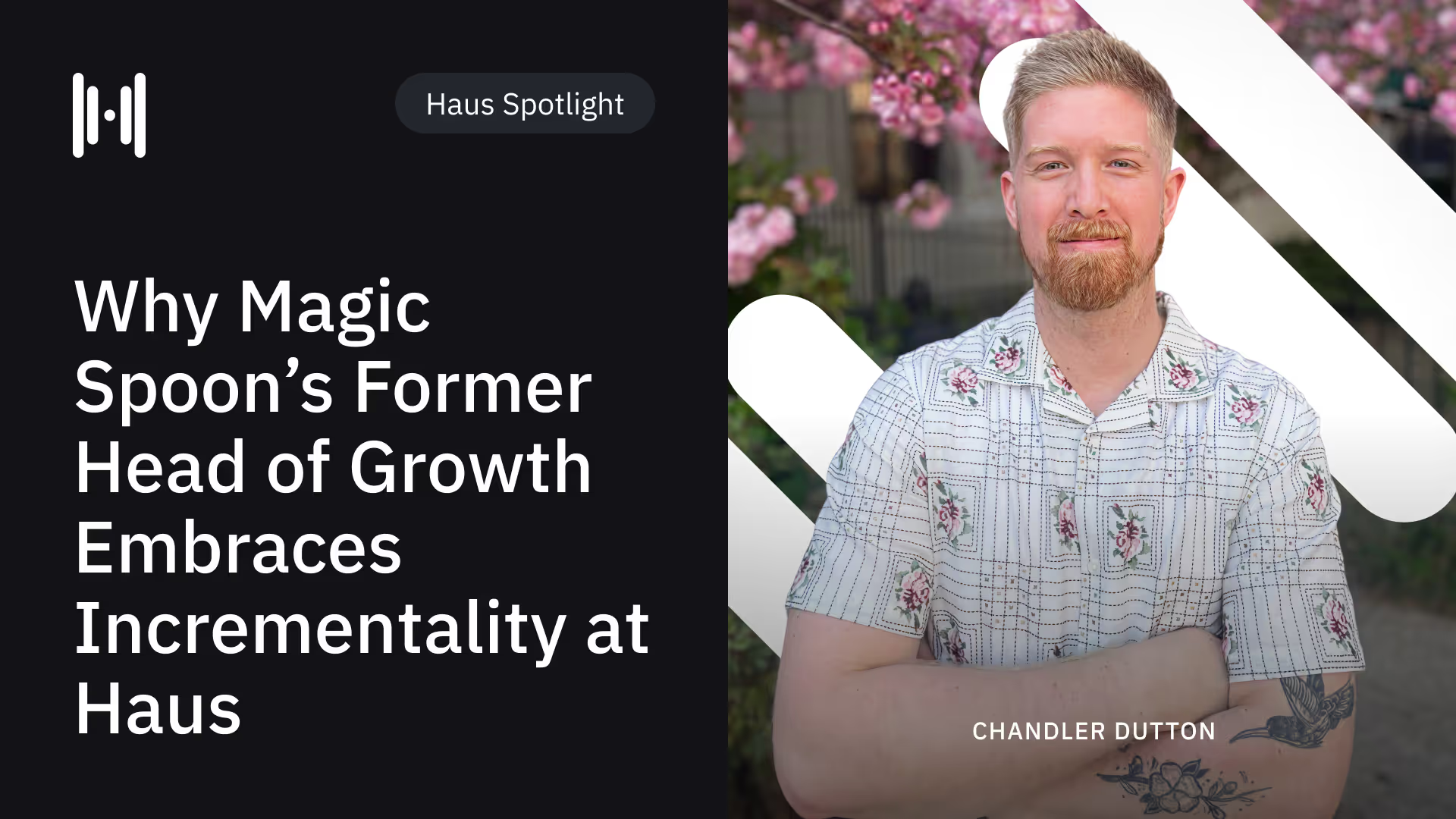
In our first episode of Haus Spotlight, we speak to Measurement Strategist Chandler Dutton about the in-the-weeds approach Haus takes with customers.
Inside the Offsite: Why Haus?
.png)
At this year's offsite, we dove into why – of all the companies, options, and career paths out there – our growing team chose Haus.
Haus Named One of LinkedIn's Top Startups
.avif)
A note from Zach Epstein, Haus CEO.
The Haus Viewpoint

We are building Haus to democratize access to world-class decision science tools.
Education
Learn the ins and outs of different marketing science concepts.
Marketing Measurement: What to Measure and Why
.png)
This guide outlines the metrics, testing methods, and proven frameworks you can use to measure marketing effectiveness in 2025.
What You’re Actually Measuring in a Platform A/B Test

Platform creative tests may not meet the definition of a causal experiment, but they can be performance optimization tool within the bounds of the algorithm.
Should I Build My Own MMM Software?
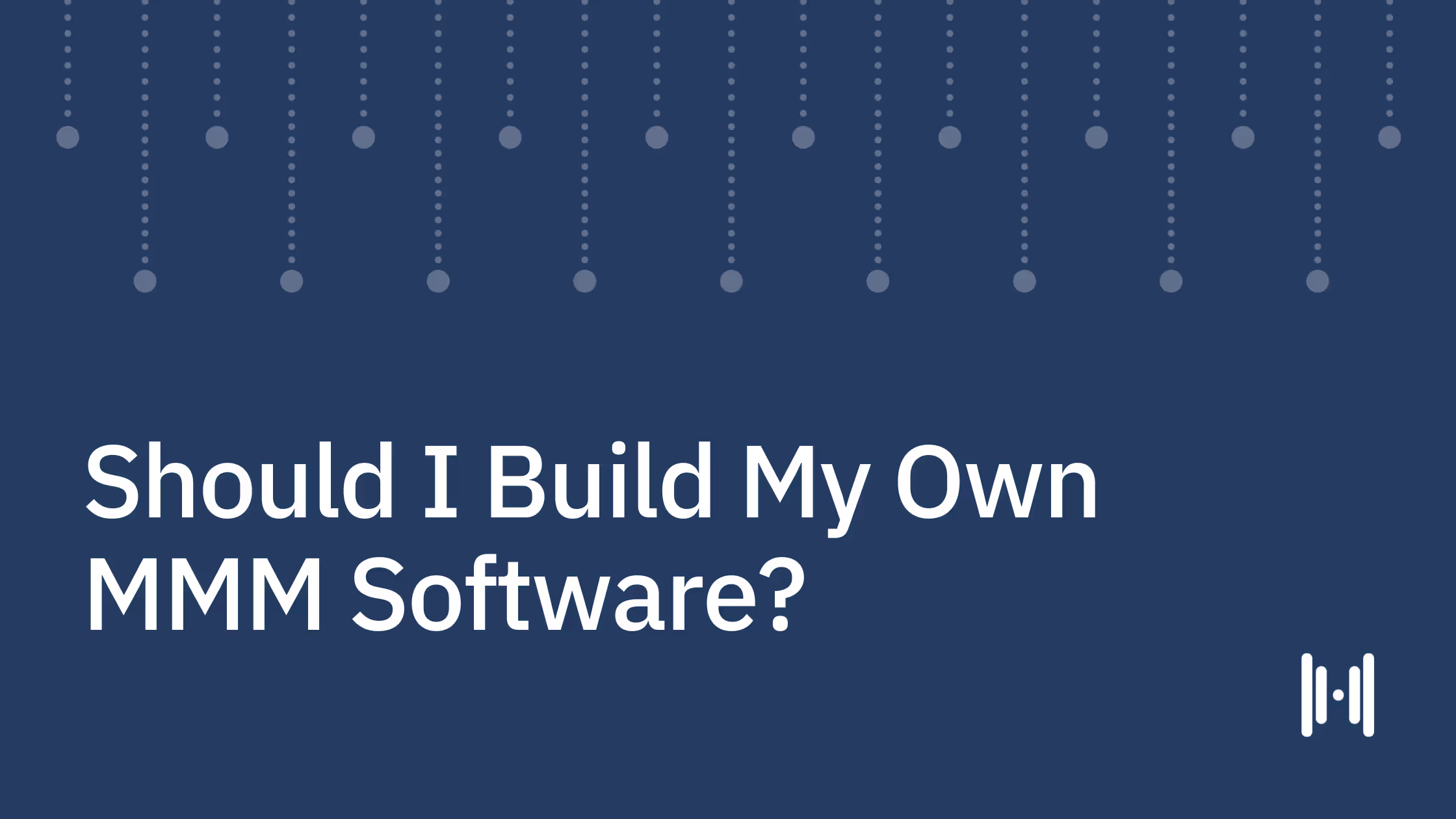
Let's unpack the pros and cons of building your own in-house marketing mix model versus working with a dedicated measurement partner.
Understanding Incrementality Testing
.avif)
Fuzzy on some of the nuances around incrementality testing? This guide goes deep, unpacking detailed examples and step-by-step processes.
MMM Software: What Should You Look For?
.avif)
We discuss some of the key questions to ask a potential MMM provider — and the importance of prioritizing causality.
How to Know If An Incrementality Test Result Is ‘Good’ – And What to Do About It
.avif)
Plus: What to do when a test result is incremental but not profitable, and a framework for next steps after a test.
Getting Started with Causal MMM
.avif)
Causal MMM isn’t rooted in historical correlational data – it’s rooted in causal reality.
Incrementality: The Fundamentals
.avif)
Let's explore incrementality from every angle — what it is, what you can test, and what you need to get started.
Getting Started with Incrementality Testing

As the customer journey grows more complex, incrementality testing helps you determine the true, causal impact of your marketing.
Incrementality School, E6: How to Foster a Culture of Incrementality Experimentation
.avif)
Having the right measurement toolkit for your business is only meaningful insofar as your team’s ability to use that tool.
How Does Traditional Marketing Mix Modeling (MMM) Work?
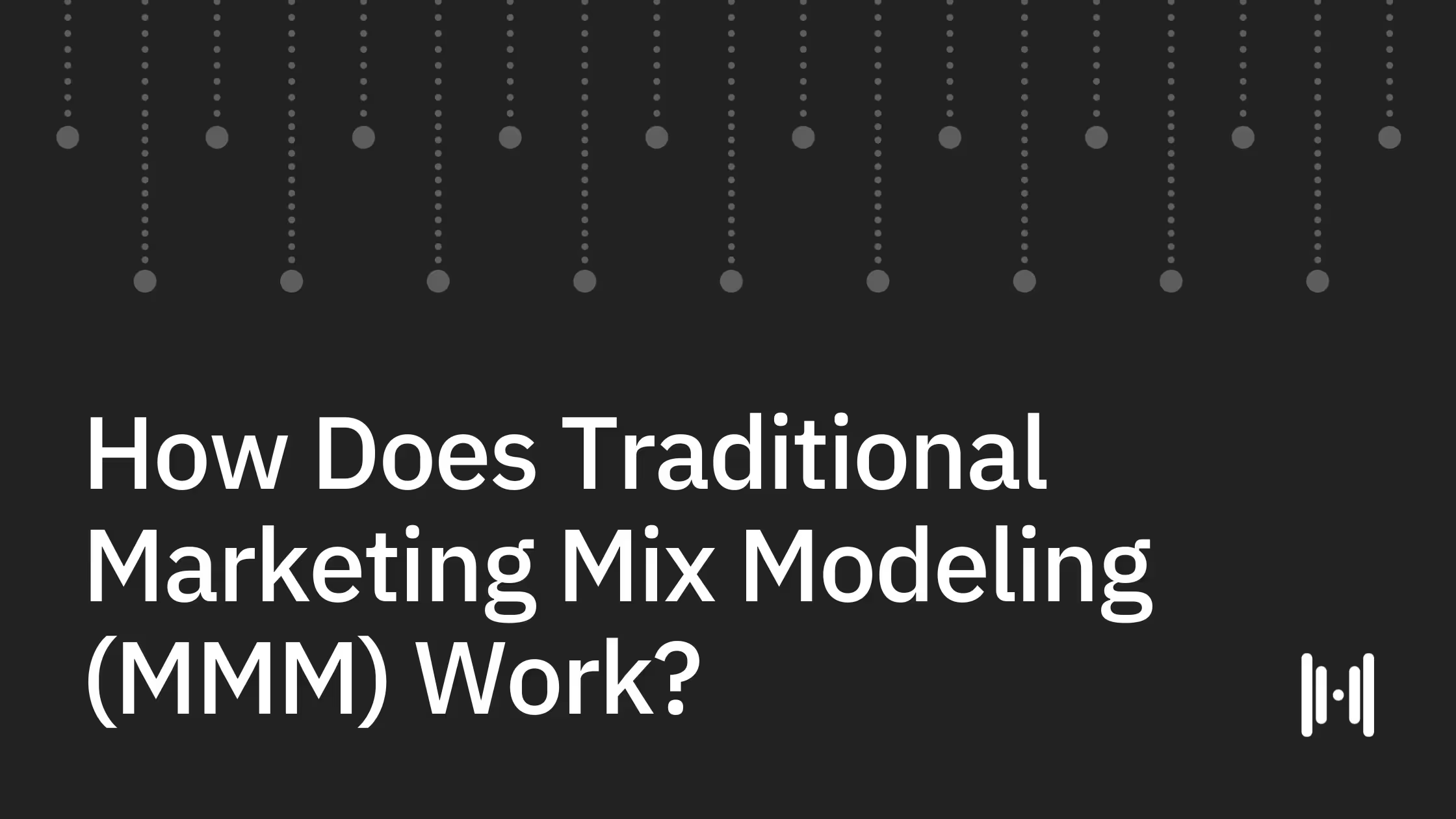
Traditional marketing mix modeling (MMM) often relies on linear regression to illustrate correlation, not causation.
Incrementality School, E5: Randomized Control Experiments, Conversion Lift Testing, and Natural Experiments
.avif)
Sure, the title's a mouthful – but attributing changes in data (ex: ‘my KPI went up') to certain factors (ex: ‘we increased ad spend’) is hard to do well.
Incrementality Testing: How To Choose The Right Platform

Whether you’re actively evaluating incrementality platforms or simply curious to learn more, consider this checklist your first stop.
Incrementality School, E4: Who Needs Incrementality Testing?

As brands' marketing strategies grow in complexity, incrementality testing becomes increasingly consequential.
Incrementality School, E3: How Do Brands Measure Incrementality?
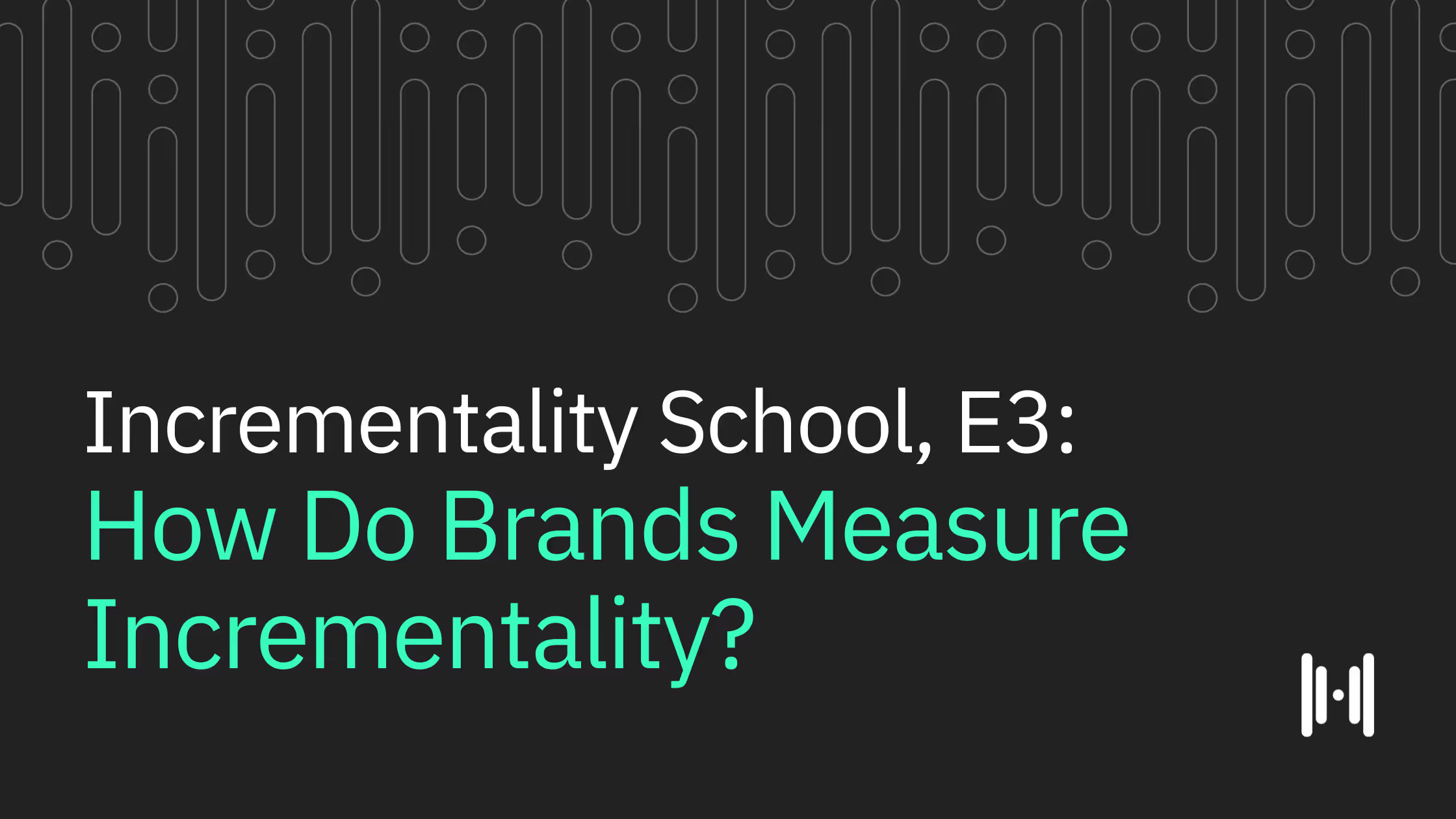
Traditional MTAs and MMMs won't measure incrementality – but geo experiments reveal clear cause, effect, and value.
Incrementality School, E2: What Can You Incrementality Test?

Haus’ Customer Marketing Lead Maddie Dault and Success Team Lead Nick Doren dive into what you can incrementality test – and why now's the time.
Incrementality School, E1: What is Incrementality?

To kick off our new Incrementality School series, three Haus incrementality experts weigh in describing a very fundamental concept.
3 Ways to Perfect Your Prime Day Marketing Strategy
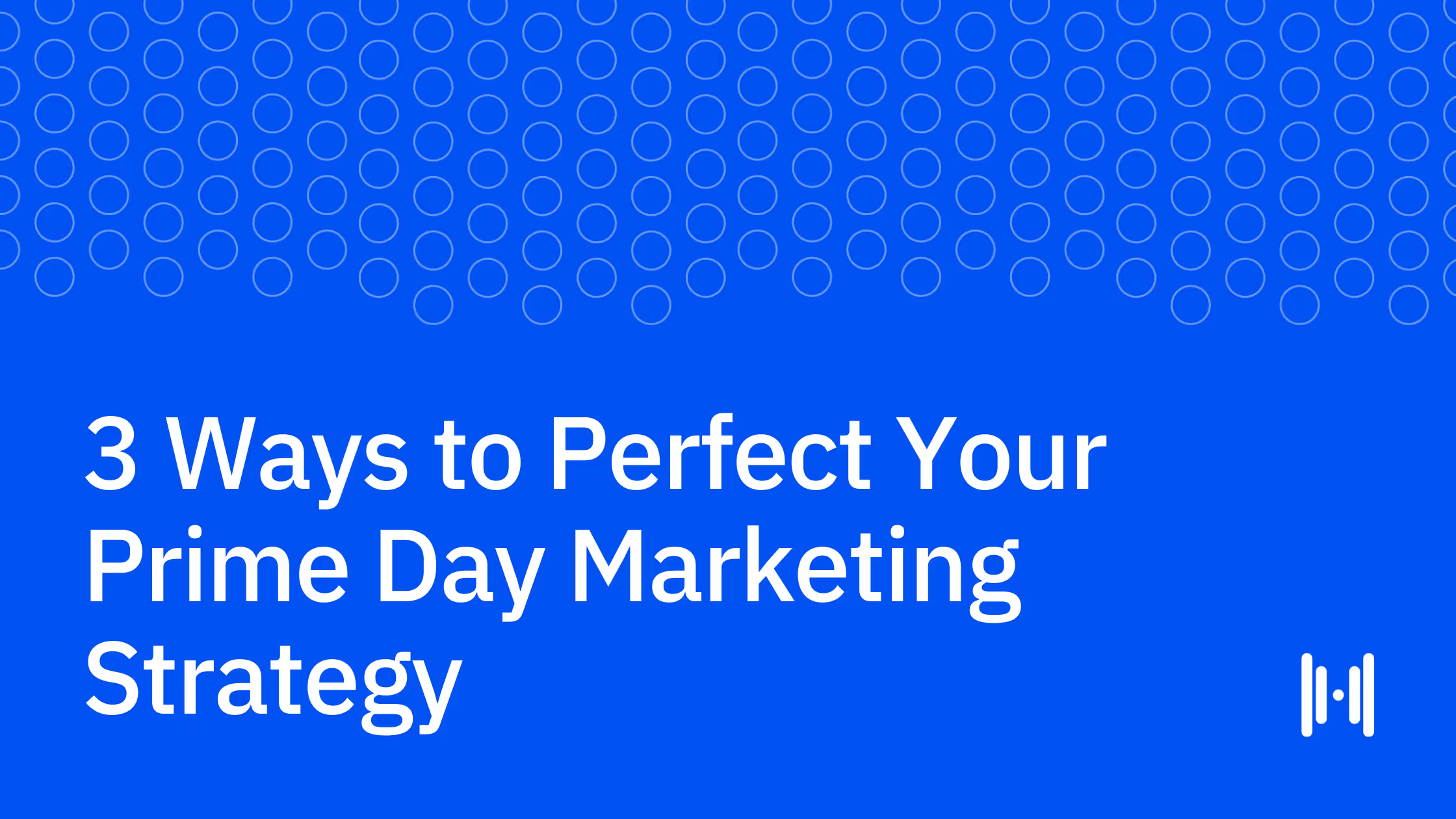
Think Amazon ads are the only effective marketing channel for Prime Day? Think again.
Maximize Your Q4 Growth With 4 High-Impact, Low-Risk Tests

Not testing during your busy season may be costing you more than you think.
Why Maturing Direct to Consumer Brands Need to Run Incrementality Tests

The media strategy that gets DTC brands from zero to one does not get them from one to ten.
5 Signs It’s Time to Invest in Incrementality
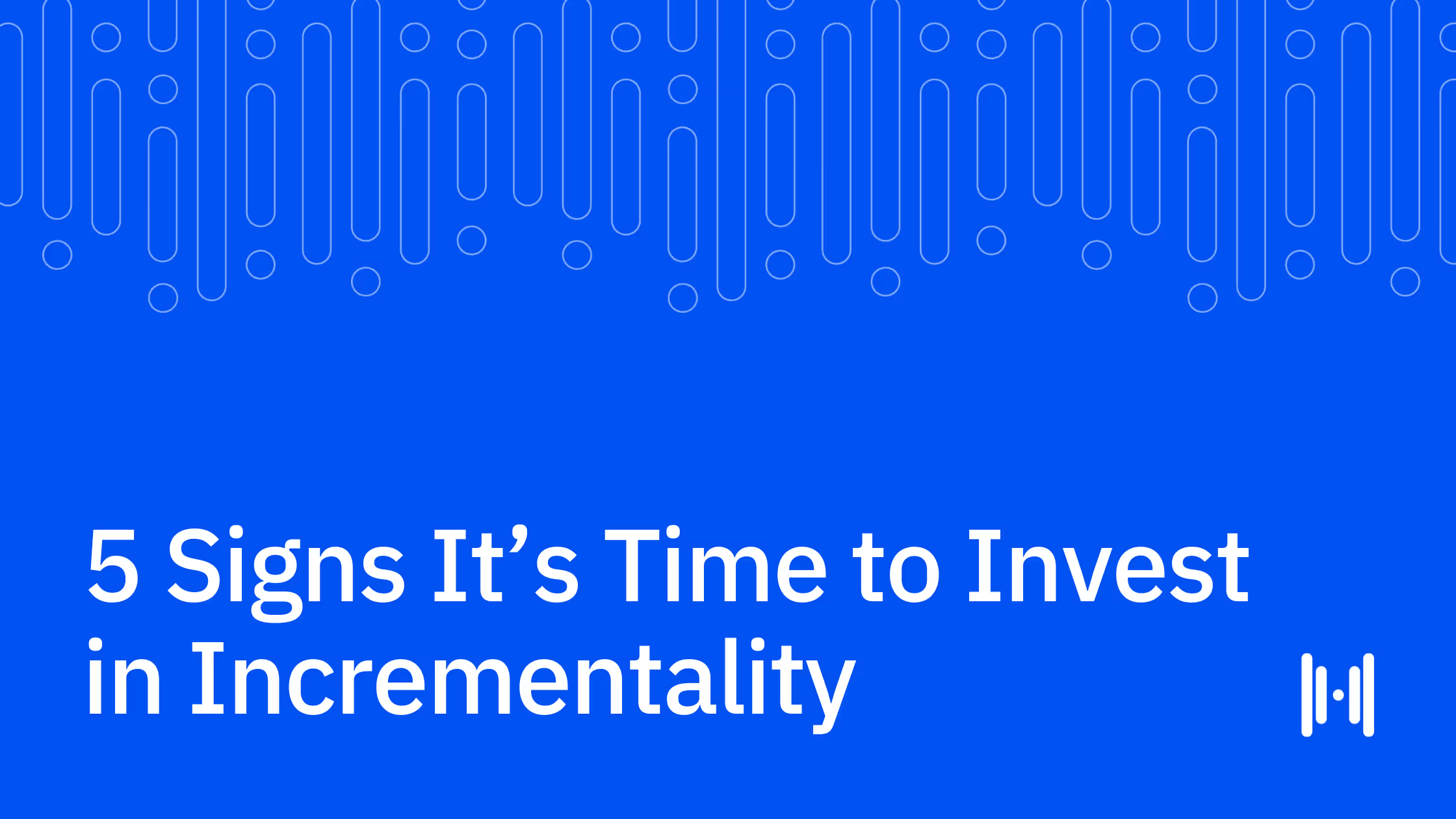
5 common signs that indicate it is definitely time to start investing in incrementality.
Why You Need a 3rd Party Incrementality Partner

Are you stuck wondering if you should be using 3rd party incrementality studies, ad platform lift studies, or trying to design your own? Find out here.
How Automation Is Transforming Growth Marketing

As platforms force more automation, the role of the media buyer is evolving. Read on to learn what to expect and what levers are left to pull.
The Secret to Comparing Marketing Performance Across Channels

While incrementality is better than relying on attribution alone, comparing them as-is is challenging. Thankfully, there’s a better way to get an unbiased data point regardless of the channel.
Your Attribution Model Is Precise but Not Accurate - Here’s Why

Learn which common marketing measurement tactics are accurate, precise, neither or both.
How to Use Causal Targeting to Save Money on Promotions

Leverage causal targeting to execute promotions that are actually incremental for your business.
Are Promotions Growing Your Business or Losing You Money?

Promotions, despite their potential power and ubiquity, are actually hard to execute well.
User-Level Attribution Is Out. Geo-Testing Is the Future.
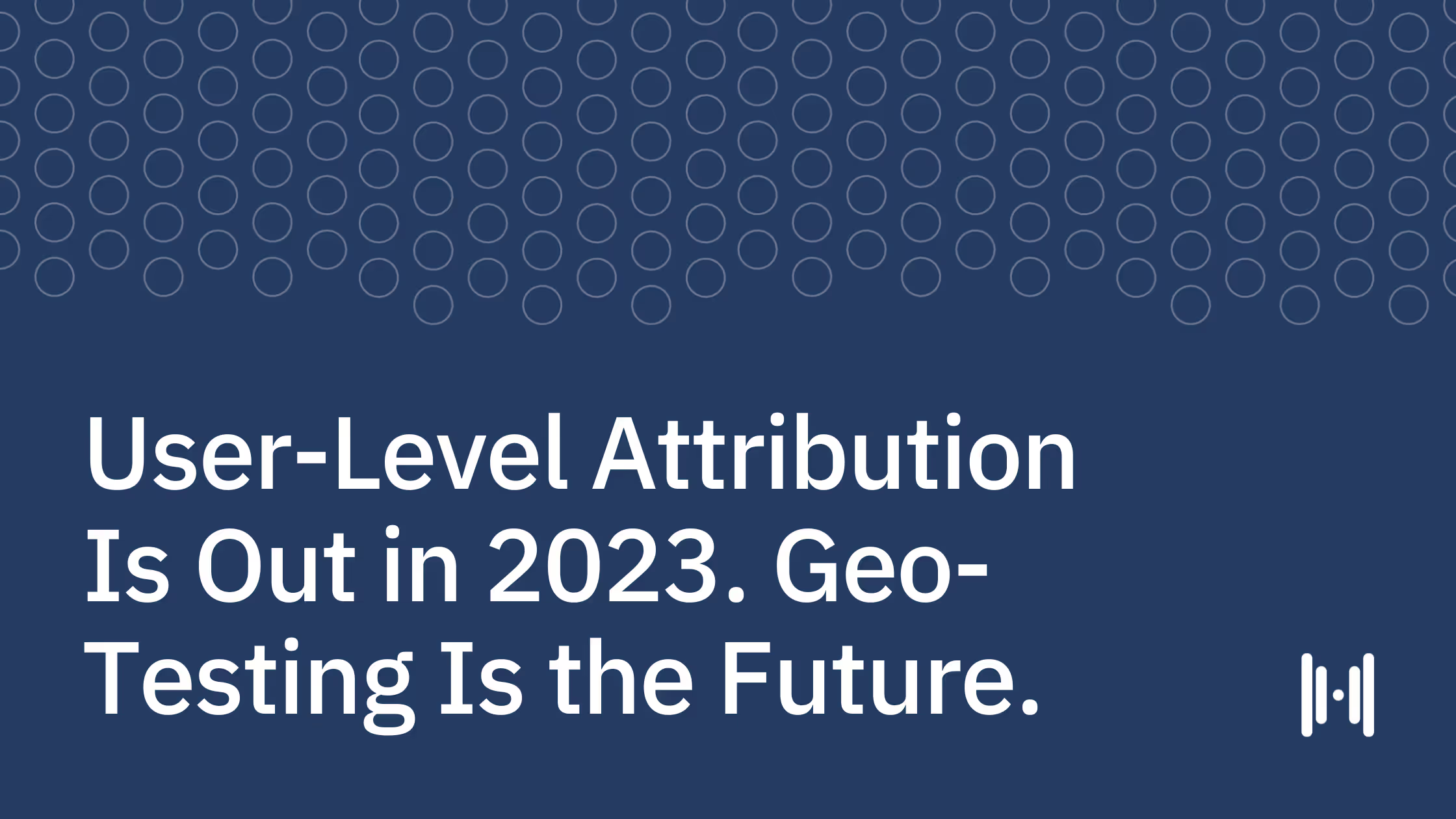
Geotesting is a near-universal approach for measuring the incremental effects of marketing across both upper and lower funnel tactics.