Incrementality Is Not Just for Acquisition
June 27, 2023
In today’s world of marketing measurement, incrementality is the hottest topic.
This popularity makes sense, since increased privacy regulations and the deprecation of cookies have made attribution more and more unreliable. Most marketers use experimentation to test acquisition channels and strategies. But we know it's typically more cost effective to retain a customer than acquire a new one - so why not apply the same framework to customer upsell and retention strategies as well? You can and should use incrementality testing to boost LTV.
In this article, we’ll jump into how to apply customer incrementality in practice.
Customer Offers
Customer offers should be optimized for incrementality or else money is being wasted.
Say it’s Black Friday and you decide to email your customers with a 30% off sitewide promotion. Now, let’s imagine 1,000 of your customers purchase a new product using the black friday promotion. Some of those customers might have needed that 30% to purchase but others might only have needed 20% off. There is likely even a handful that were going to purchase anyway and happened to score a great deal. By giving all your customers a blanket discount, you likely wasted money when you didn’t have to.
This is why incrementality is important for customer promotions! If you can identify which of your customers need the promotion to drive incremental action and how generous that promotion needs to be, you can maximize the efficiency of a sale.
Personalization
This same concept can be applied to non-monetary customer strategies as well. For example, you can determine which customers are most likely to take action from an email versus SMS or who might need both forms of outreach to take an action. Taking a personalized approach can help you avoid churn with the customers who don’t want to be bothered with frequent communication too.
There are tons of customer touch points that can be personalized. Amazon tends to be king in this department, using hundreds of customer data points to personalize each experience. One of the lead Applied Scientists that created Amazon’s personalization engine now works at Haus and is democratizing this practice for all brands via the CustomerLift product.
Customer Incrementality in Practice
Customer incrementality testing with causal ML is a new notion and even more nuanced and complex than regional geo-experiments, which is likely why most brands aren’t doing this in-house.
When running a standard A/B test we aspire to estimate the Average Treatment Effect (ATE), which is essentially the difference between the averages in the treatment and control groups. However, to do causal targeting we need to estimate the effect of the treatment on each individual, which is aptly called Individualized Treatment Effect (ITE).
To start, you must run an initial randomized test of your treatment. Then, you create and train a machine learning (ML) model utilizing customer level data and outcomes from that initial test. The ML model will provide specific treatments for particular customer segments based on your budget considerations, max number of people targeted, and any other guardrails you wish to include. You will then implement those treatments with each designated customer cohort. The more you run these tests and feed the results back into your model, the better and more accurate the model becomes.
As you can imagine, creating a model is intricate and requires considerable expertise which is why we created our CustomerLift product. Haus will build your causal machine learning model for you and provide you with all the automation and tools to train it appropriately. Then, you can use your ML model as frequently as you’d like to test any customer treatments you want to optimize. CustomerLift makes customer incrementality and personalization easy for all brands.
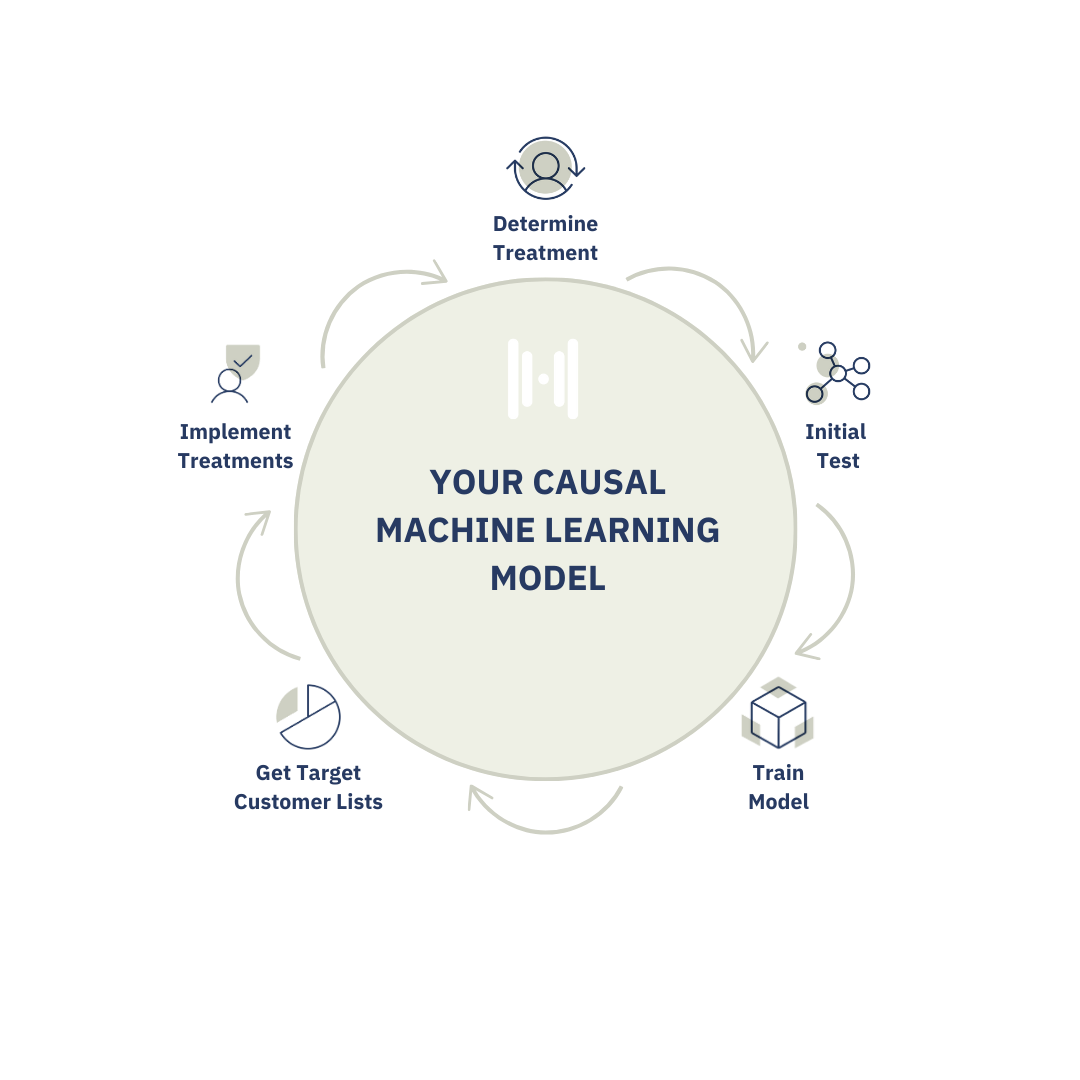
Ready to see Haus’s CustomerLift product in action? Book a demo today.